Yang Lu joined the Cheriton School of Computer Science as an Assistant Professor in 2023. Previously, he was a postdoctoral researcher in Professor William Noble’s genome sciences group at the University of Washington. He obtained his PhD in Computational Biology and Bioinformatics under the supervision of Professor Fengzhu Sun at the University of Southern California. Before moving to the United States, he completed his MS and BS in Computer Science and Engineering from Shanghai Jiao Tong University in China.
Yang leads the BATMEN — BioinformAtics & Trustworthy Machine lEarNing — Lab, a research group based at the Cheriton School of Computer Science. He and his students develop interpretable machine learning models to make sense of complex biological data and discover scientifically interesting and statistically confident hypotheses by interpreting these models.
The following is a lightly edited transcript of a Q and A interview.
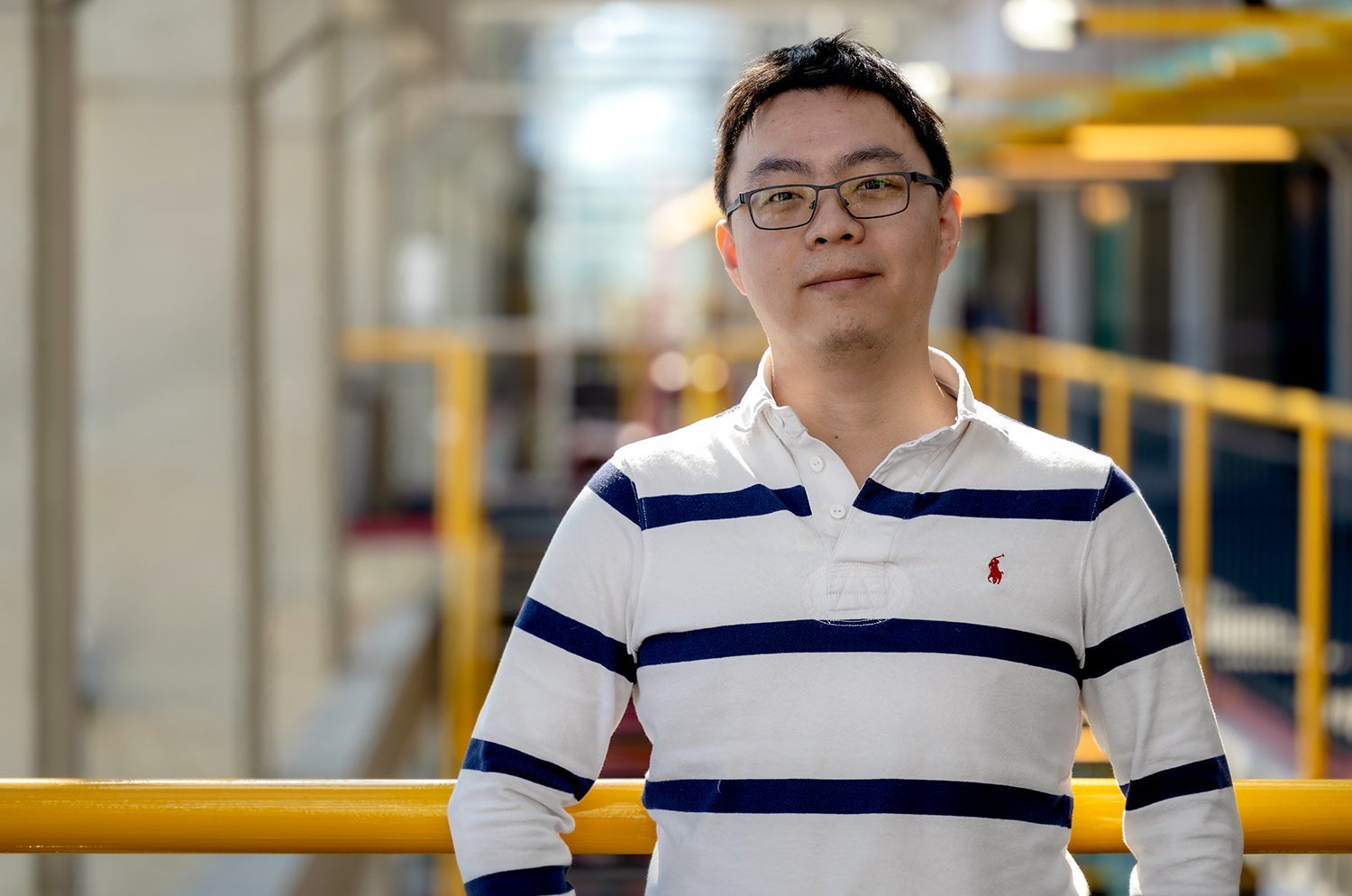
Tell us a bit about yourself.
My career path is a bit different from other faculty in computer science in that I was trained as a software engineer as an undergraduate, but then took an interesting detour. When I graduated with my bachelor’s degree my plan was to become a software engineer, but during my master’s degree I tried something different that changed my path.
I did a lot of internships during my undergraduate degree, and I was getting burned out. But I also wanted to try something different as I’ve long believed that computer science itself is not as powerful as computer science plus X. In my case, that X was biology.
I went to the United States to study computational biology, a program that combined algorithmic computer science with statistics and biology. I joined the Department of Genome Sciences at the University of Washington, a purely biology department. I learned the language biologists speak, while identifying and working on interesting computational problems that computer scientists are trained to solve.
When I searched for a faculty position, I switched back to computer science as I believe that’s the discipline where I can maximize my expertise across a range of biological problems.
What led to your interest in bioinformatics?
I have experience and interest in a wide range of biological problems, but I come to them from the perspective of a computer scientist.
Let me draw an analogy between biology and computer science. Living things have a source code known as the genome, the set of genes or genetic material in a cell or an organism. This source code is compiled by a compiler using an intermediary file, which in biological systems corresponds to RNA. In other words, the genetic material — DNA — is transcribed to an intermediary molecule called RNA. This intermediary file is ultimately turned into an executable file. In biology, the executable file is the translation of RNA into a protein molecule.
Once an executable file runs, there will be some sort of log or trace to indicate that it has been executed. In biology, that trace is metabolic activity. When a protein molecule does some function in a cell, energy is consumed and metabolic products leave a trace that can be analyzed.
My interest in bioinformatics stems from this hierarchy in biology.
What attracted you to the Cheriton School of Computer Science?
Joining the School of Computer Science was both an easy and hard decision for me. It was easy because the School is large and prestigious with many experts, making it easy to find collaborators with skills that complement mine. Professors Ming Li and Bin Ma, for example, are world-renown bioinformatics researchers who I knew even before I applied for a faculty position here. Joining the School where they are faculty was an easy decision.
But the hard part was deciding whether to stay in the United States or to make another international move, this time to Canada. That’s not an easy decision, but Bin Ma convinced me to join the School of Computer Science. It’s also great that Canada is welcoming of immigrants, both as professionals and as citizens.
Tell us a bit about your research.
In a general sense, biologists are trying to find a needle in a haystack. For example, of the tens of thousands of biomarkers, which ones are useful to diagnose a disease, develop treatments or determine prognosis? Biologists have traditionally approached this problem in a laborious and tedious way — by posing a hypothesis then collecting data to test it.
Researchers in biology and medicine have collected an unprecedented amount of data from a variety of sources. My research builds upon the benefits of extracting information from such big data sources by building a so-called automatic hypothesis-generation machine, where the input is the big data people have collected. The outputs are the hypotheses deduced by artificial intelligence or machine learning techniques that biologist might be interested in with high confidence.
For example, we have much data on the behaviour of genes and proteins in cancer patients and in healthy people. We want to make predictions to differentiate between cancer patients and healthy people and we do this by interrogating a machine learning model to give us some knowledge into the genes or gene networks that might explain why some people get cancer and others do not.
Do you see opportunities for collaborative research with colleagues at the School of Computer Science?
Yes, my research area is broad and overlaps with every faculty member in the Bioinformatics Group. I’m already co-supervising students with Professors Bin Ma and Lila Kari.
But I’m also a member of the AI and Machine Learning Group. I’ve talked to Professor Wenhu Chen to discuss the possibility of using natural language processing models such as ChatGPT to revolutionize how we deal with biological data. What if we used ChatGPT as an interface that lets scientists talk to the data? I’ve also met with Professors Pascal Poupart and Yaoliang Yu about using AI and machine learning to solve biological problems, then applying the methods developed to solve problems in other domains.
What do you see as your most important or most significant contribution?
One of my most impactful research contributions is in deep learning. Deep learning is exceptionally good at capturing subtle relationships in large data sets that experts themselves may not see. But it may be hard to convince scientists, clinicians and doctors that deep learning networks are very good at some task when we do not know how the system made its decision. The problem is that although the performance of a deep learning system may be exceptionally good, it’s like a black box that does not reveal why and consequently may not be trusted.
My work — in a paper titled DeepPINK: Reproducible Feature Selection in Deep Neural Networks — is the first that demonstrated that the interpretation a deep neural network system can achieve a statistical guarantee. This research is important not just because it was the first work to demonstrate a statistical guarantee, but also because it attracted many follow-up studies by experts at top research universities.
Who has inspired you most?
My postdoctoral advisor — William Noble at the University of Washington — was a great inspiration. He is an exceptional researcher, but what I benefited from most was his ability to manage a research group with 20 to 30 people effortlessly and his ability to encourage us to work hard without feeling pressured.
That kind of management skill greatly benefitted me, and I think it will continue to do so when I manage my own research group.
What do you do in your spare time?
I spend a lot of time with my son. In a way, raising a child is like an observational study — a child comes into the world without knowledge and understanding, but over time learns to conceive the world and its complexities. I also enjoy watching football, or soccer as it’s called here.