PhD candidate Vasisht Duddu, Intel Labs research scientist Sebastian Szyller, and Professor N. Asokan have been honoured with a Distinguished Paper Award for their work titled “SoK: Unintended Interactions among Machine Learning Defenses and Risks.” Their paper was presented at the 45th IEEE Symposium on Security and Privacy, the premier forum for showcasing developments in computer security and electronic privacy.
“Congratulations to Vasisht, Asokan, and their colleague Sebastian on receiving a distinguished paper award,” said Raouf Boutaba, University Professor and Director of the Cheriton School of Computer Science. “Although considerable research has been conducted on security and privacy risks in machine learning models, further work is needed to understand how specific defences interact with other risks. Their award-winning systematization of knowledge paper offers a framework to identify and explain interactions between defences and risks, allowing them to conjecture about unintended interactions.”
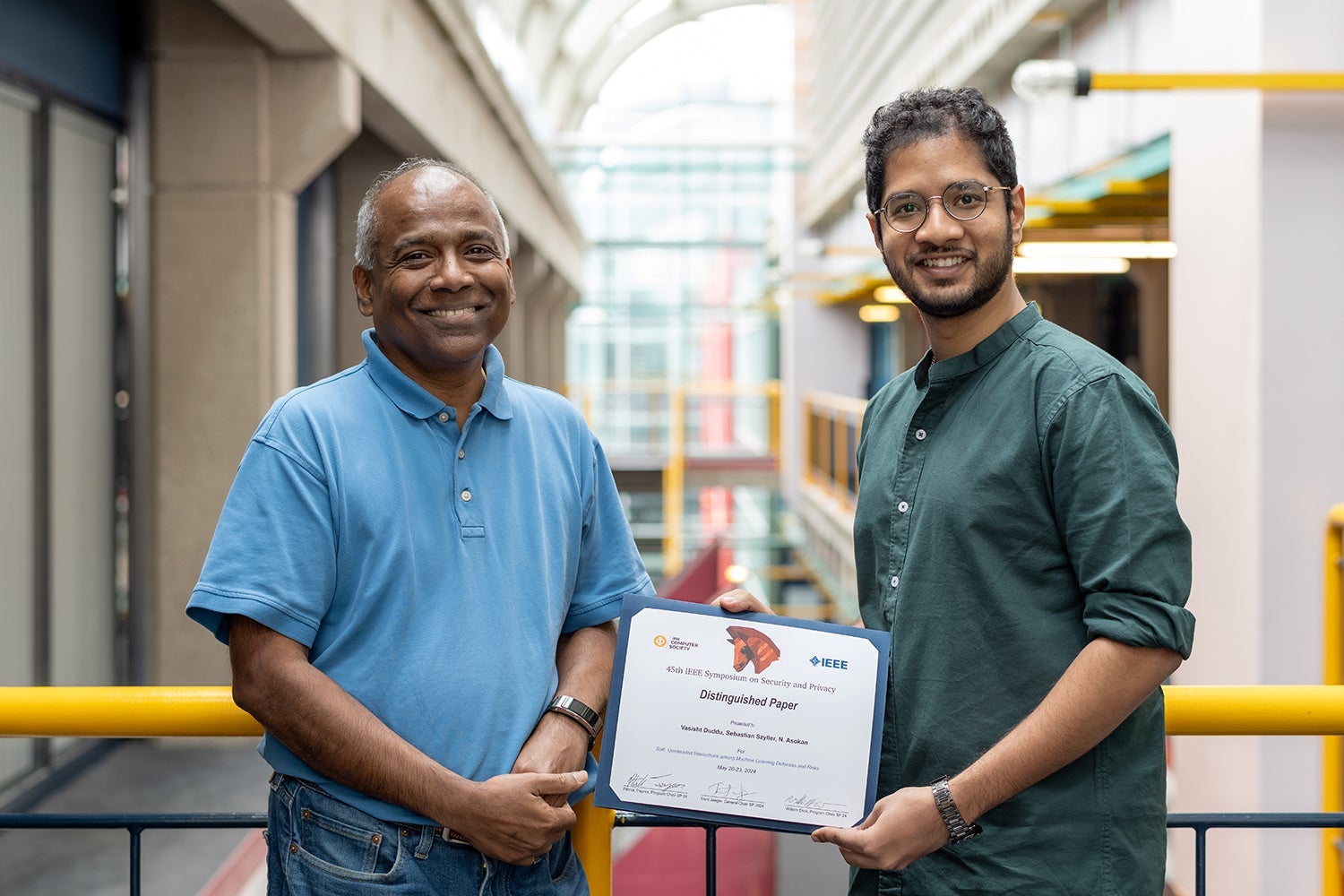
L
to
R:
Professor
N.
Asokan
and
PhD
candidate
Vasisht
Duddu.
Sebastian
Szyller
was
unavailable
for
the
photo.
Vasisht
Duddu
is
pursuing
a
PhD
at
the
Cheriton
School
of
Computer
Science.
His
research
focuses
on
risks
to
security,
privacy,
fairness,
and
transparency
in
machine
learning
models.
He
also
designs
attacks
to
exploit
these
risks
and
defences
to
counter
them
to
better
understand
the
interplay
between
risks
and
defences.
Additionally,
he
works
on
ensuring
accountability
in
machine
learning
pipelines
to
meet
regulatory
requirements.
N.
Asokan
is
a
Professor
of
Computer
Science
at
Waterloo
where
he
holds
a
David
R.
Cheriton
Chair
and
serves
as
the
Executive
Director
of
the
Waterloo
Cybersecurity
and
Privacy
Institute.
Asokan’s
primary
research
theme
is
systems
security
broadly,
including
topics
like
developing
and
using
novel
platform
security
features,
applying
cryptographic
techniques
to
design
secure
protocols
for
distributed
systems,
applying
machine
learning
techniques
to
security
and
privacy
problems,
and
understanding
and
addressing
the
security
and
privacy
of
machine
learning
applications
themselves.
Sebastian
Szyller
is
a
research
scientist
at
Intel
Labs.
He
works
on
various
aspects
of
security
and
privacy
of
machine
learning.
Recently,
he
has
been
working
on
model
extraction
attacks
and
defences,
membership
inference,
and
differential
privacy.
More
broadly,
he
is
interested
in
different
ways
to
protect
machine
learning
models
and
data
to
enable
robust
and
privacy-preserving
analysis,
both
in
terms
of
the
technical
details
as
well
as
legislation
compliance.
Sebastian
was
a
visiting
PhD
student
at
Waterloo
during
fall
2022.
More about this award-winning research
Machine learning models are vulnerable to a variety of risks to their security, privacy and fairness. Although various defences have been proposed to protect against risks individually, when a defence is effective against one risk it may inadvertently lead to an increased susceptibility to other risks. Adversarial training to increase robustness, for example, also increases vulnerability to membership inference attacks.
Predicting such unintended interactions is challenging. A unified framework that clarifies the relationship between defences and risks can help researchers identify unexplored interactions and design algorithms with better trade-offs. It also helps practitioners to account for such interactions before deployment. Earlier work, however, was limited to studying a specific risk, defence or interaction as opposed to systematically studying their underlying causes. A comprehensive framework spanning multiple defences and risks to systematically identify potential unintended interactions is currently absent.
The research team addressed this gap by systematically examining various unintended interactions across multiple defences and risks. They hypothesized that overfitting and memorization of training data are the potential causes underlying these unintended interactions. An effective defence may induce, reduce or depend on overfitting or memorization, which in turn affects the model’s susceptibility to other risks.
Their study identified several factors — such as the characteristics of the training dataset, its objective function, and the model — that collectively influence a model’s propensity to overfit or memorize. These factors provide insight into understanding the susceptibility to different risks when a defence is employed.
Key contributions of the study
- Developed the first systematic framework to understand unintended interactions by their underlying causes and factors that influence them
- Conducted a comprehensive literature survey to identify different unintended interactions, situating them within the framework, and a guideline to the framework to hypothesize about unintended interactions
- Identified previously unexplored unintended interactions for future research, using the framework to hypothesize two such interactions and empirically validating them
For further details about this award-winning research, please see the paper: Vasisht Duddu, Sebastian Szyller, N. Asokan. SoK: Unintended Interactions among Machine Learning Defenses and Risks, 2024 IEEE Symposium on Security and Privacy, San Francisco, CA, 2024.
Research group’s project page: https://ssg-research.github.io/mlsec/interactions
Blog article: https://blog.ssg.aalto.fi/2024/05/unintended-interactions-among-ml.html