A team of theoretical neuroscientists has received the European Neural Network Society Best Paper Award at ICANN 2024, the 33rd International Conference on Artificial Neural Networks. The prestigious recognition was given for their paper “Biologically-plausible Markov Chain Monte Carlo Sampling from Vector Symbolic Algebra-encoded Distributions.”
Led by P. Michael Furlong, Research Officer at the NRC-UW Collaboration Centre, along with colleagues Kathryn Simone, Nicole Dumont, Madeleine Bartlett, Terrence Stewart and Professors Jeff Orchard and Chris Eliasmith, the work describes a way that a network of spiking neurons can generate random samples from a probability distribution. The distribution is encoded using vector symbolic algebra, a type of compositional language embedded in a vector space.
“How do brains represent probability distributions and sample from them to make decisions, plan into the future, or imagine different scenarios?,” said Nicole Dumont, a PhD candidate at the Cheriton School of Computer Science. “Models of such functions should be able to sample different types of structures, not just numbers but things such as trajectories through a space like your office building, sequences of actions, and even language.”
Vector symbolic algebras provide a unifying framework for representing these different data structures and more, all in a high-dimensional vector space, using the properties of distributed representations.
“We introduced an algorithm for sampling from distributions represented in a particular vector symbolic algebra,” Dr. Simone explains. “It enables us to sample different kinds of data structures all using the same recurrent neural network architecture. We like this approach because it provides a generalizable neural circuit that could explain behaviour in the face of uncertainty for many kinds of tasks.” Dr. Furlong continued, “It would make sense that the brain would have a decision-making mechanism that can work in many different scenarios, instead of just one circuit per decision. We demonstrated that capability by sampling over a few different data structures. In our future work, we will include more complex structures, like language.”
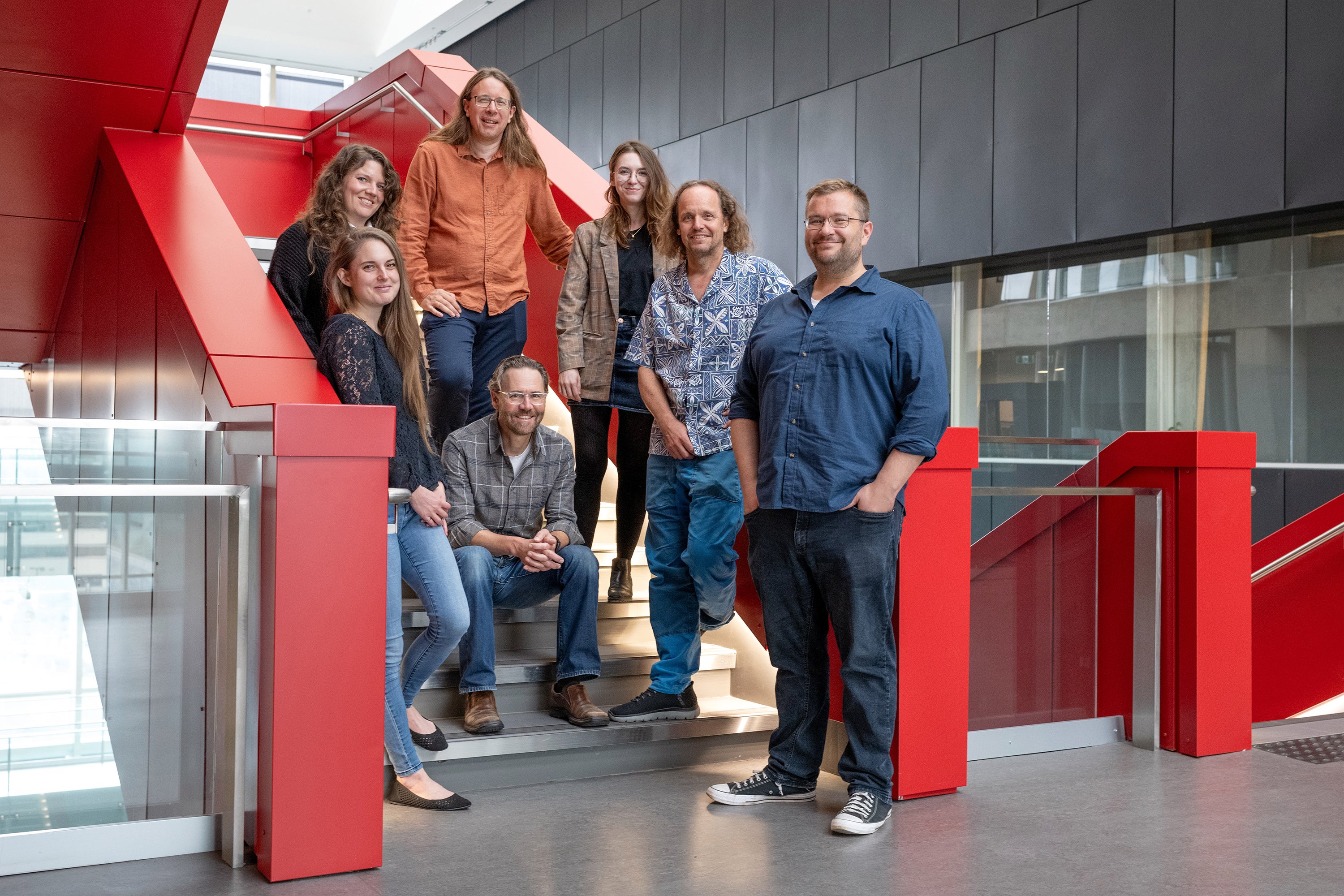
Front (L to R): Kathryn Simone, Professor Jeff Orchard (sitting), P. Michael Furlong; Back (L to R): Maddy Bartlett, Terry C. Stewart, Nicole Dumont, Professor Chris Eliasmith
Dr. Kathryn Simone is a Postdoctoral Fellow in Waterloo’s Neurocognitive Computing Lab. She works on biologically plausible reinforcement learning methods and neural novelty detection.
Dr. Maddy Bartlett is a Postdoctoral Fellow in Waterloo’s Neurocognitive Computing Lab. Her research applies new neural processing strategies to reinforcement learning, and models of the basal ganglia.
Dr. Terry Stewart is a Research Officer at the National Research Council. He is a co-founder of Applied Brain Research, a research-based start-up based on using neuromorphic computer chips and adaptive neural algorithms. He is one of the researchers behind Spaun, the first biologically realistic brain simulation that can perform multiple tasks.
Nicole Dumont is a PhD candidate advised by Professors Jeff Orchard and Chris Eliasmith. Her research involves developing advanced neurocognitive modelling techniques and their application in reinforcement learning and navigation.
Jeff Orchard is an Associate Professor at the Cheriton School of Computer Science, and director of the Neurocognitive Computing Lab. His research goal is to find something akin to the theory of evolution for the brain — that is, a set of simple mechanistic rules that govern the development and operation of the brain, resulting in cognition and complex behaviours.
Chris Eliasmith is a Professor appointed jointly in the Departments of Philosophy and Systems Design Engineering. He is the founding director of the Centre for Theoretical Neuroscience, a focal point for researchers across Waterloo’s Faculties of Mathematics, Engineering, Arts and Science who are interested in computational and theoretical models of neural systems.
Dr. P. Michael Furlong is a Research Officer at the NRC-UW Collaboration Centre. His research interests span probability modelling, active learning, and neurorobotics. He previously worked in automating planetary exploration as a KBR contractor at NASA Ames’ Intelligent Robotics Group.
More about this award-winning research
Managing uncertainty is essential for organisms, as the ability to encode, manipulate and sample probabilistic information about the environment is necessary to handle uncertainty that arises in perception and decision-making. However, the neurological basis of the mathematical descriptions of probability and uncertainty in brains remains poorly understood.
Previous approaches to modelling probability in neural systems have proposed methods that map random variables onto individual neurons. Another approach proposes that populations of neurons represent distributions over random variables, and allows biologically plausible sampling through linear decoding of values from neural activity. Nonetheless, a gap persists between mathematical probabilistic cognitive models and actual implementation by neurons.
A promising way to bridge this gap is to use vector symbolic algebras — a family of algebras over high-dimensional vector spaces. These algebras provide a hypothesis about the structure of the latent representations that neural networks manipulate to perform cognitive tasks. One such algebra is the holographic reduced representation algebra, and more recent restricted forms to represent continuous data called spatial semantic pointers. Inherently probabilistic, spatial semantic pointers offer insights into how organisms might reason about uncertainty, but the ability to sample from these probabilistic representations — critical for decision-making models — remains understudied.
The researchers hypothesized that the holographic reduced representation algebra has advantages over the kernel mean embedding formulation for implementation in resource-constrained neural networks. Specifically, the algebra preserves dimensionality, bounding resource requirements for representing arbitrary compositions of data, as well as provides a computationally simple method for conditioning distributions.
The vector symbolic algebra that the researchers use to embed distributions strongly resembles techniques developed for kernel mean embeddings. Sampling from vector embeddings of distributions has been explored in the context of the kernel mean embedding literature, but these methods rely on internal knowledge of the feature-space encoding in the form of gradients.
Accessing this knowledge neurologically is problematic for two reasons: First, it is hypothesized that the brain operates on cognitive representations that are compressions of the enormous volume of data that comes in through one’s senses. Sampling from that underlying space would require maintaining huge repositories of sensory information with high fidelity — an expensive and improbable proposition for brain activity. Second, relying on the gradient demands maintaining information about the neural circuits that the brain uses to turn sensory information into the compressed, cognitive representation. If this were the case, then the brain would not only need to know how to represent data, but it would also need a representation of how that representation is produced, which would increase the real estate required for neural circuitry considerably.
Consequently, turning vector symbolic algebra-encoded distributions into specific decisions or actions requires a method for sampling from those distributions directly.
To this end, the researchers explored Hamiltonian Monte Carlo sampling from the vector symbolic algebra-encoded distributions using Langevin dynamics. Langevin dynamics are particularly interesting because they can be implemented by the dynamics of recurrent neural networks, and because Monte Carlo sampling has been proposed as an explanation for how brains can be probabilistic yet still diverge from optimal decision-making.
The key contribution of their research is development of a biologically plausible sampler for distributions encoded using the holographic reduced representation algebra. They argue that this approach is biologically plausible. First, the vector symbolic algebra adopted as their feature space embedding was developed in the context of cognitive modelling and is used to implement models that can be readily translated into neural networks, capable of reproducing physiological data. Second, by not relying on knowledge of the gradient of the encoding scheme, they avoid the cost of representing the gradient in neurons, on top of the representations themselves. Third, the use of Langevin dynamics adds biological plausibility since they are readily implemented by neural networks.
These biological advantages extend beyond cognitive modeling. They also have practical implications for neuromorphic hardware, where they can offer improved energy efficiency.
To learn more about the research on which this article is based, please see P. Michael Furlong, Kathryn Simone, Nicole Sandra-Yaffa Dumont, Madeleine Bartlett, Terrence C. Stewart, Jeff Orchard, Chris Eliasmith. Biologically-plausible Markov Chain Monte Carlo Sampling from Vector Symbolic Algebra-encoded Distributions. In: M Wand, K Malinovská, J Schmidhuber, IV Tetko (eds) Artificial Neural Networks and Machine Learning – ICANN 2024. ICANN 2024. Lecture Notes in Computer Science, vol 15019. Springer, Cham.
This work was funded by CFI and OIT infrastructure funding, Canada Research Chairs program, NSERC Discovery grant 261453, NUCC NRC File A-0028850, AFOSR grant FA9550-17-1-0026, and a gift from the Intel Corporation.