Maura R. Grossman, JD, PhD, is a Research Professor in the Cheriton School of Computer Science, an Adjunct Professor at Osgoode Hall Law School, and an affiliate faculty member of the Vector Institute for Artificial Intelligence. She is also Principal at Maura Grossman Law, an eDiscovery law and consulting firm in Buffalo, New York.
Maura is best known for her work on technology-assisted review, a supervised machine learning approach that she and her colleague, Computer Science Professor Gordon V. Cormack, developed to expedite review of documents in high-stakes litigation.
She teaches Artificial Intelligence: Law, Ethics, and Policy, a course for graduate computer science students at Waterloo and upper-class law students at Osgoode, as well as the ethics workshop required of all students in the master’s programs in artificial intelligence and data science at Waterloo.
The following is a lightly edited transcript of a Q&A interview.
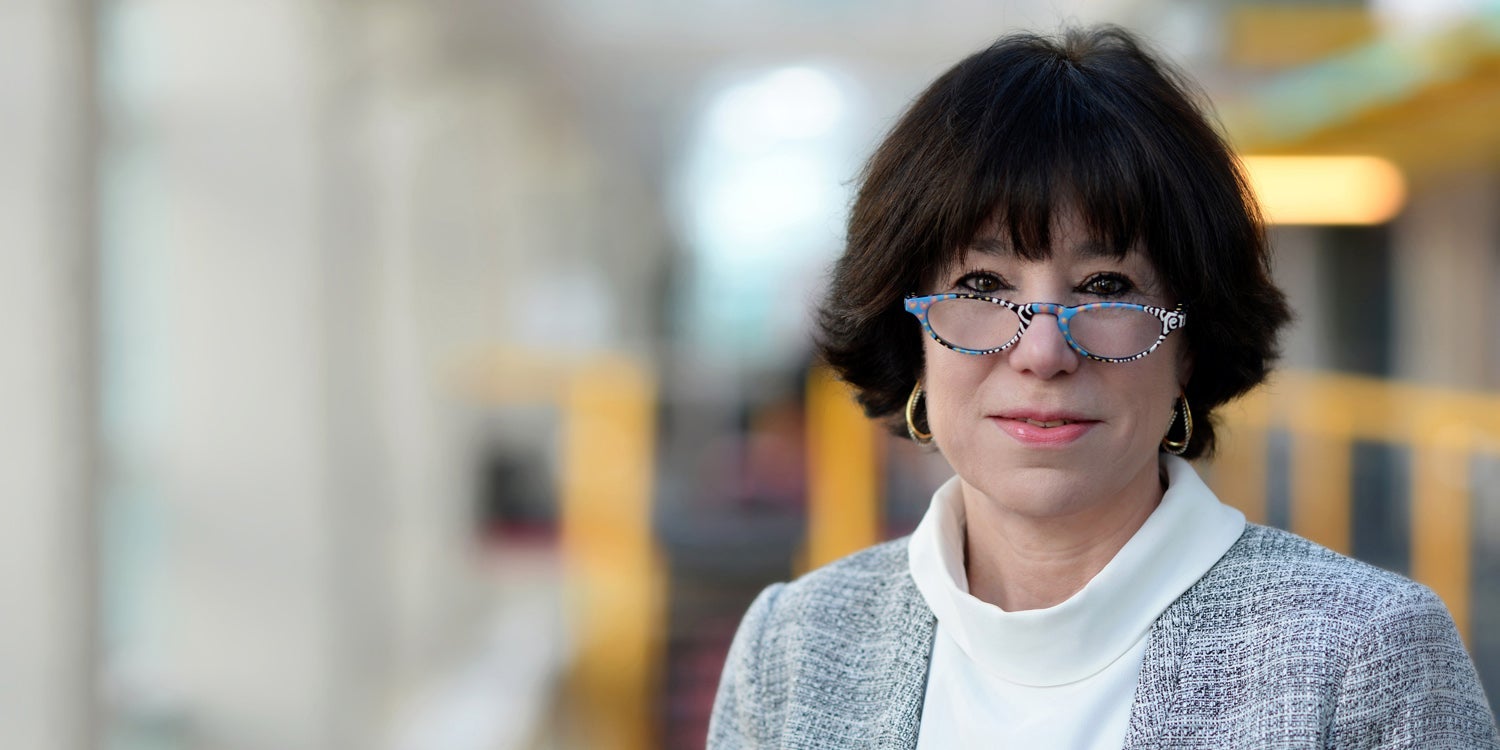
What is AI?
Artificial intelligence is an umbrella term first used at a conference in Dartmouth in 1956. AI means computers performing cognitive tasks — such as thinking, reasoning, and predicting — that were once thought to be the sole province of humans. It’s not a single technology or function.
Generally, AI involves algorithms, machine learning, and natural language processing. An algorithm is simply a sequence of precise rules to solve a problem or perform a task.
There are basically two types of AI, though some people believe there are three. The first is narrow or weak AI. This kind of AI does some task at least as well as, if not better than, a human. We have AI technology today that can read an MRI more accurately than a radiologist can. In my field of law, we have technology-assisted review — AI that can find legal evidence more quickly and accurately than a lawyer can. Other examples are programs that play chess or AlphaGo better than top players.
The second type is general or strong AI; this kind of AI would do most if not all things better than a human could. This kind of AI doesn’t yet exist, and there’s debate about whether we’ll ever have strong AI. The third type is super intelligent AI, and that’s really more in the realm of science fiction. This type of AI would far outperform anything humans could do across many areas. It’s obviously controversial though some see it as an upcoming existential threat.
Why are we hearing so much about AI now?
AI has become prominent because of a confluence of factors. The first is the exponential growth in computational power. We carry more computing power in our pockets today than what NASA had to perform the calculations needed to land humans on the moon.
The second is that we have more data than ever. For one, we have the Internet, and the Internet allows us to collect and access massive amounts of data that are needed to train AI algorithms. The third is the development of open source software communities that have lowered the barrier to entry. If you want to build some kind of AI you can go to an open source site such as GitHub, download code that does something similar to the intended task, and with a bit of modification, start using it right away.
How is AI different from conventional computer programming?
In the past, if you wanted to program a computer you had to write down all the steps in the right order and then you had to translate them into instructions using a programming language. Now, we have machine learning, where we give large amounts of data to an algorithm and it can figure out the rules itself. This allows us to do many things that would be time consuming — if not impossible — were we to attempt them by programming a computer step by step.
There are different kinds of machine learning. Unsupervised machine learning is a kind of AI where you do not train the computer with labelled data or tell it what you’re looking for. Instead, the AI looks for patterns, clusters, groupings, and anomalies, which were previously unknown to you.
Another type is supervised machine learning. If I want to train a computer to distinguish between pictures of puppies and kittens, I can give it labelled examples — this is a picture of a puppy, this is a picture of a kitten. The algorithm learns what features distinguish a puppy from a kitten. Once trained, you can give the computer an unlabelled picture and it can determine if it’s a photo of a puppy or a kitten. Supervised learning systems infer mathematical functions or rules from the old data to make predictions about new data.
Yet another type of AI is reinforcement learning. Say I don’t have training examples to give the computer beforehand and I want to train the AI as I go. I can teach the algorithm using positive and negative reinforcement and it will learn as it goes. Reinforcement learning finds a balance between exploration and exploitation, where exploration goes into new territory and exploitation goes deeper into something we already know.
Deep learning, another type of AI, uses multiple layers of algorithms that transform complex input into mathematical representations. For example, with speech recognition, the AI system might begin with digitized electrical signals, the next layer might be phonemes, followed by words, then phrases and parts of speech. All of this information would be combined at the upper levels to make predictions about meaning. We can do much more complex tasks with deep learning, but often we don’t know what’s happening underneath the hood — what features of the data are being used and how they’re weighted — so deep learning is less explainable and transparent than other algorithms, but it’s also more powerful.
There’s also natural language processing or NLP. NLP tries to understand human language as it’s written or spoken by making a computer representation of the language, including both its syntax and semantics. While a supervised machine learning algorithm designed to distinguish between puppies and kittens does not care about meaning, Siri or Alexa do care about something resembling meaning. Question answering requires something resembling an understanding of meaning. Translation between languages requires something resembling an understanding of meaning.
Where is AI being used?
AI is used in countless areas.
In healthcare, AI is used to detect tumours in MRI scans, to diagnose illness, and to prescribe treatment. In education, AI can evaluate teacher performance. In transportation, it’s used in autonomous vehicles, drones, and logistics. In banking, it’s determining who gets a mortgage. In finance, it’s used to detect fraud. Law enforcement uses AI for facial recognition. Governments use AI for benefits determination. In law, AI can be used to examine briefs parties have written and look for missing case citations.
AI has become interwoven into the fabric of society and its uses are almost endless.
What is ethical AI?
AI isn’t ethical, just as a screwdriver or a hammer isn’t ethical. AI may be used in ethical or unethical ways. What AI does, however, is raise several ethical issues.
AI systems learn from past data and apply what they have learned to new data. Bias can creep in if the old data that’s used to train the algorithm is not representative or has systemic bias. If you’re creating a skin cancer detection algorithm and most of the training data was collected from White males, it’s not going to be a good predictor of skin cancer in Black females. Biased data leads to biased predictions.
How features get weighted in algorithms can also create bias. And how the developer who creates the algorithm sees the world and what that person thinks is important — what features to include, what features to exclude — can bring in bias. How the output of an algorithm is interpreted can also be biased.
How has AI been regulated, if at all?
Most regulation so far has been through soft law — ethical guidelines, principles, and voluntary standards. There are thousands of soft laws and some have been drafted by corporations, industry groups, and professional associations. Generally, there’s a fair degree of consensus as to what would be considered proper or acceptable use of AI — for example, AI shouldn’t be used in harmful ways to perpetuate bias, AI should have some degree of transparency and explainability, it should be valid and reliable for its intended purpose.
The most comprehensive effort to date to generate a law to govern AI was proposed in April 2021 by the European Union. This draft EU legislation is the first formal AI regulation. It classifies AI into risk categories. Some uses of AI are considered unacceptably high risk and they tend to be things like using AI to manipulate people psychologically. Another prohibited use is AI to determine social scores, as in the People’s Republic of China, where a person is monitored and gets points for doing something desirable and loses points if doing something undesirable. A third prohibited use is real-time biometric surveillance.
The next category is high-risk AI tools like those used in medicine and self-driving vehicles. A company must meet all sorts of requirements, conduct risk assessments, keep records, and so on before such AI can be used. Then there are low-risk uses, such as web chatbots that answer questions. Such AI requires transparency and disclosure, but not much else.
Is there a place for education about law in computer science and for computer science education in law?
Unquestionably, and this is something I address in my courses.
Developers, in general, don’t have much background in law and policy. Say a computer scientist is asked to create an AI system and the data used to train it is biased in some way. The person might think it’s the data scientist’s problem because this is where the data came from. The computer scientist may believe their role is only to create and optimize the algorithm. But the computer scientist might be the only person who has any knowledge about the data, how it’s been collected and cleansed, and may be the only person who is in a position to do anything about the problem.
On the other hand, law students go on to write laws and regulations, as lawyers, policy makers, and politicians, but they typically have little understanding of the technology, so they may propose blunt instruments, or draft regulations that have unintended consequences.
You have this disconnect where few people have both skills. That’s why I teach courses that cross disciplinary boundaries. And it goes well beyond those two disciplines. You need a multiplicity of stakeholders to talk about the ethical issues, not just people with a background in law and computer science. A diversity of viewpoints and expertise is critical in many areas, but especially in applying ethics to artificial intelligence.
Can AI conform to human values or social expectations?
It’s very difficult to train an algorithm to be fair if you and I cannot agree on a definition of fairness. You may think that fairness means the algorithm should treat everyone equally. I might believe that fairness means achieving equity or making up for past inequities.
Our human values, cultural backgrounds, and social expectations often differ, leaving it difficult to determine what an algorithm should optimize. We simply don’t have consensus yet.
In machine learning, we often don’t know what the system is doing to make decisions. Are transparency and explainability in AI important?
That’s a difficult question to answer. There is definitely something to be said for transparency and explainability, but in many circumstances it may be good enough if the AI has been tested sufficiently to show that it works for its intended purpose. If a doctor prescribes a drug, the biochemical mechanism of action may be unknown, but if the medication has been proven in clinical trials to be safe and effective, that may be enough.
Another way to look at this is, if we choose to use less sophisticated AI that we can more easily explain, but it is not as accurate or reliable than a more opaque algorithm, would that be an acceptable tradeoff? How much accuracy are we willing to give up in order to have more transparency and explainability?
It may depend on what the algorithm is being used for. If it’s being used to sentence people, perhaps explainable AI matters more. In other areas, such as identifying tumors, accuracy is the more important criterion. It comes down to a value judgment.