Linda Wang (she/her), a third-year Computer Science student, is the Co-op Student of the Year for the Faculty of Math! Her incredible work at the Royal Bank of Canada (RBC) played a pivotal role in developing one of the company’s first generative Artificial Intelligence (GenAI) use cases in human resources (HR).
Linda discusses her proudest accomplishments, the challenges of applying AI in industry and the importance of stepping outside of your comfort zone.
During her co-op term at the Royal Bank of Canada (RBC), Linda Wang utilized AI technologies to develop a solution for the Global HR Advice Center, now pending patent, to reshape internal HR inquiry processes for over 90,000 employees.
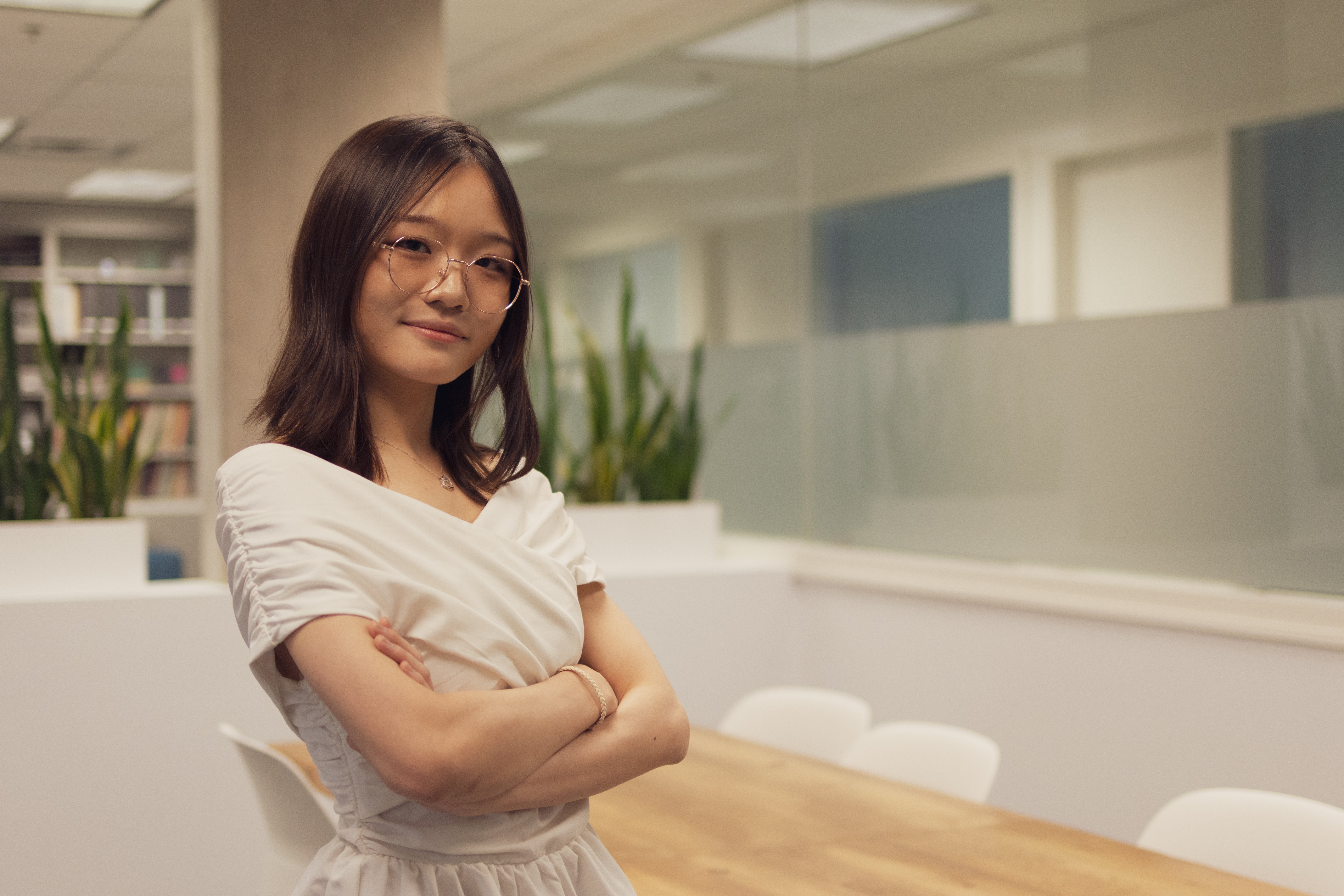
Traditional HR processes often require significant time and resources, creating bottlenecks in large organizations.
To address this challenge, Linda designed an AI-powered system that enhances efficiency by streamlining responses to employee inquiries.
Her work introduced one of RBC’s first GenAI applications in HR, a field where AI adoption has been relatively slow. By implementing this solution, she not only improved operational efficiency but also demonstrated how AI can drive innovation beyond client-facing applications.
Where do you feel you made the biggest impact in your time at RBC?
The solution that I developed last summer marked one of the first GenAI use cases in the HR department, which I believe is one of the more underrepresented departments, not only at RBC, but in the industry overall
The newest technology tends to favour client-side applications, not necessarily HR, but I like to say that HR is the backbone of every organization.
How has co-op shaped or changed your understanding of the AI industry?
Being able to apply AI in the real world is very different from research.
For example, one of the biggest differences I noticed was that everything is generally open to imagination in research, but in industry, there are many more constraints. So, you have to learn that done is better than perfect.
Time and cost are always a huge constraint in a business setting because oftentimes you're trying to save money. So, you can't, for example, spend billions of dollars developing a solution even though it might save billions.