The following excerpt is from “The quest for high-quality data: Machine learning solutions for data integration, cleaning, and data generation are beginning to emerge,” a blog post written by Cheriton School of Computer Science Professor Ihab Ilyas and Ben Lorica, Chief Data Scientist at O’Reilly Media.
“AI starts with ‘good’ data” is a statement that receives wide agreement from data scientists, analysts, and business owners. There has been a significant increase in our ability to build complex AI models for predictions, classifications, and various analytics tasks, and there’s an abundance of (fairly easy-to-use) tools that allow data scientists and analysts to provision complex models within days.
As model building become easier, the problem of high-quality data becomes more evident than ever. A recent O’Reilly survey found that those with mature AI practices (as measured by how long they’ve had models in production) cited “Lack of data or data quality issues” as the main bottleneck holding back further adoption of AI technologies.
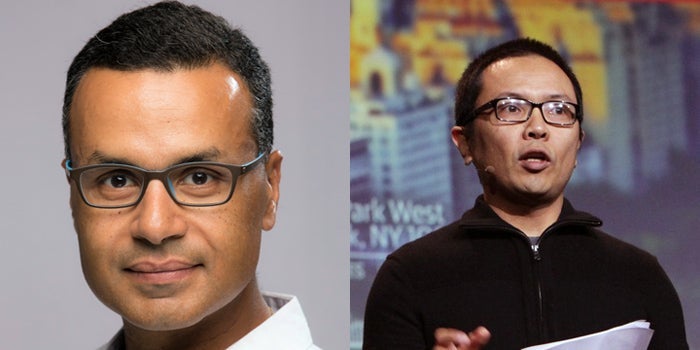
L to R: Professor Ihab Ilyas and Ben Lorica
Even with advances in building robust models, the reality is that noisy data and incomplete data remain the biggest hurdles to effective end-to-end solutions. The problem is even more magnified in the case of structured enterprise data. These data sets are often siloed, incomplete, and extremely sparse. Moreover, the domain knowledge, which often is not encoded in the data (nor fully documented), is an integral part of this data (see this article from Forbes). If you also add scale to the sparsity and the need for domain knowledge, you have the perfect storm of data quality issues.
In this post, we shed some light on various efforts toward generating data for machine learning (ML) models. In general, there are two main lines of work toward that goal: (1) clean the data you have, and (2) generate more data to help train needed models. Both directions have seen new advances in using ML models effectively, building on multiple new results from academia.
Ihab Ilyas is a Professor in the Cheriton School of Computer Science and the Thomson Reuters-NSERC Research Chair on data quality at the University of Waterloo. His main research focuses on the areas of big data and database systems, with special interest in data quality and integration, managing uncertain data, machine learning for data curation, and information extraction. Ihab is also a co-founder of Tamr, a startup focusing on large-scale data integration. He is a recipient of the Ontario Early Researcher Award, a Cheriton Faculty Fellowship, an NSERC Discovery Accelerator Award, and a Google Faculty Award, and he is an ACM Distinguished Scientist. Ihab is an elected member of the VLDB Endowment board of trustees, elected SIGMOD vice chair, and an associate editor of the ACM Transactions of Database Systems. He has a PhD in computer science from Purdue University.
Ben Lorica is the Chief Data Scientist at O’Reilly Media, Inc. and is the Program Director of both the Strata Data Conference and the Artificial Intelligence Conference. He has applied business intelligence, data mining, machine learning and statistical analysis in a variety of settings including direct marketing, consumer and market research, targeted advertising, text mining, and financial engineering. His background includes stints with an investment management company, internet startups, and financial services.