Businesses and institutions are drenched in data and sources of data. For some large organizations, data sources number into the thousands and the data within them can be in any number of formats. This creates mind boggling challenges — from accessing and managing data to integrating, analyzing and abstracting useful information from it.
Unfortunately, the problem is poised to become more complicated. With the growing popularity of smart phones, tablets, cloud storage and devices connected to the Internet, reconciling multiple and disparate volumes of data — big data as it’s known — will become increasingly difficult.
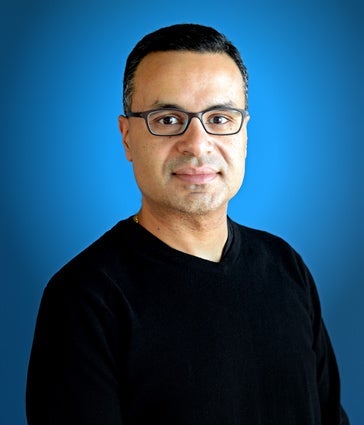
Over the last few decades, computer scientists invented various database management models to sort and manage data in files and data structures. In its early days, these systems dealt mostly with limited amounts of clean data. Amassing ever larger volumes of data was relatively easy, but accessing, managing and reasoning about the data became progressively more difficult — and this growing mismatch created a problem. In a sense computer scientists became a victim of their own success.
Ilyas’s research focuses on methods to unify increasingly large and diverse data from sources that are often dirty and inconsistent.
“Data come from many sources, and they often describe the same things in different ways,” he said. “But data can also have discrepancies and contradictions. To be able to use such data meaningfully you need to be able to reason about these discrepancies and contradictions.”
The assumptions in the early days of data management made solutions to the problem reasonably straightforward — it’s a small amount of data, the data is clean, metadata is available, so it’s all about matching. But in the current big and messy data era, data cleaning and integration have taken centre stage.
“Today data is rarely clean so we have to deal with it probabilistically rather than assuming it’s a collection of facts. So, we assume the data is a collection of observations, and the facts — whatever they are — are out there for us to discover.”
This is more difficult than it may seem, but recent advancements in machine learning have helped speed up the process.
“We begin by training a machine-learning model by giving it lots of examples of things that are the same and lots of examples of things that are not the same,” he explains. “We then let the model figure out a way to judge two new things it hasn’t encountered before and determine the probability that they are the same.”
As the term implies, the machine learns by doing and in the process becomes more accurate.
“Most machine learning is based on probability theory to reason about the likelihood of some event happening — for example, this particular event is likely, but this other event is unlikely,” Ilyas explained. “The machine chooses the more likely options and weeds out impossible ones, those that are highly improbable and so on, pruning the space of possible facts.”
The goal is to create tools and deeper understanding of data curation and abstraction that can be repeatable across many sectors.
“We’d like to transform the field of data curation and cleaning from a kitchen sink of best practices to libraries, tools and a better understanding to drive the economy more efficiently,” he said. “We can accelerate the data science part of that tenfold if we remove the overhead of cleaning and preparing data, which often consumes 90% of the time.”
Such advances will have a pronounced impact across sectors and in such diverse areas as pharmaceuticals and life sciences, financial services and industrial manufacturing. The techniques Ilyas and his team have developed have recently been applied to systematic reviews, a type of literature review that collects, critically analyzes and synthesizes results from multiple research studies.
“Conducting a thorough systematic review can be a lengthy process, often taking a year or more to search a database of literature, harvest key results, organize them, understand the biases in studies and decipher the findings,” he said. “With better and faster systematic reviews, researchers will avoid mistakes, find trends faster and consolidate results earlier, accelerating the pace of scientific discovery.”
In Tamr, a start-up cofounded by Ilyas and collaborators, the clustering, cleaning and integration techniques the team has developed have reduced the time taken to integrate and unify large amounts of data across silos by an order of magnitude.
“We cut the time to conduct large enterprise data integration projects from more than six months to just a couple of weeks,” Ilyas said. “When consolidating spending data of a large Fortune 500 company, the savings realized were in the hundreds of millions of dollars.”