Researchers at the Cheriton School of Computer Science are incorporating a deep learning network into a more accurate method to identify disease biomarkers. The new method achieves up to 98 per cent detection of peptide features in a dataset. That means scientists and medical practitioners have a greater chance of discovering possible diseases through tissue sample analysis.
Multiple techniques can detect diseases by analyzing the protein structure of bio-samples. Computer programs increasingly play a part in this process by examining the large amount of data produced in such tests to pinpoint specific markers of disease.
“But existing programs are often inaccurate or can be limited by human error in their underlying functions,” said Fatema Tuz Zohora, a PhD candidate supervised by University Professor Ming Li. “What we’ve done in our research is to create a deep neural network that achieves 98 per cent detection of peptide features in a dataset. We’re working to make disease detection more accurate to provide healthcare practitioners with the best tools.”
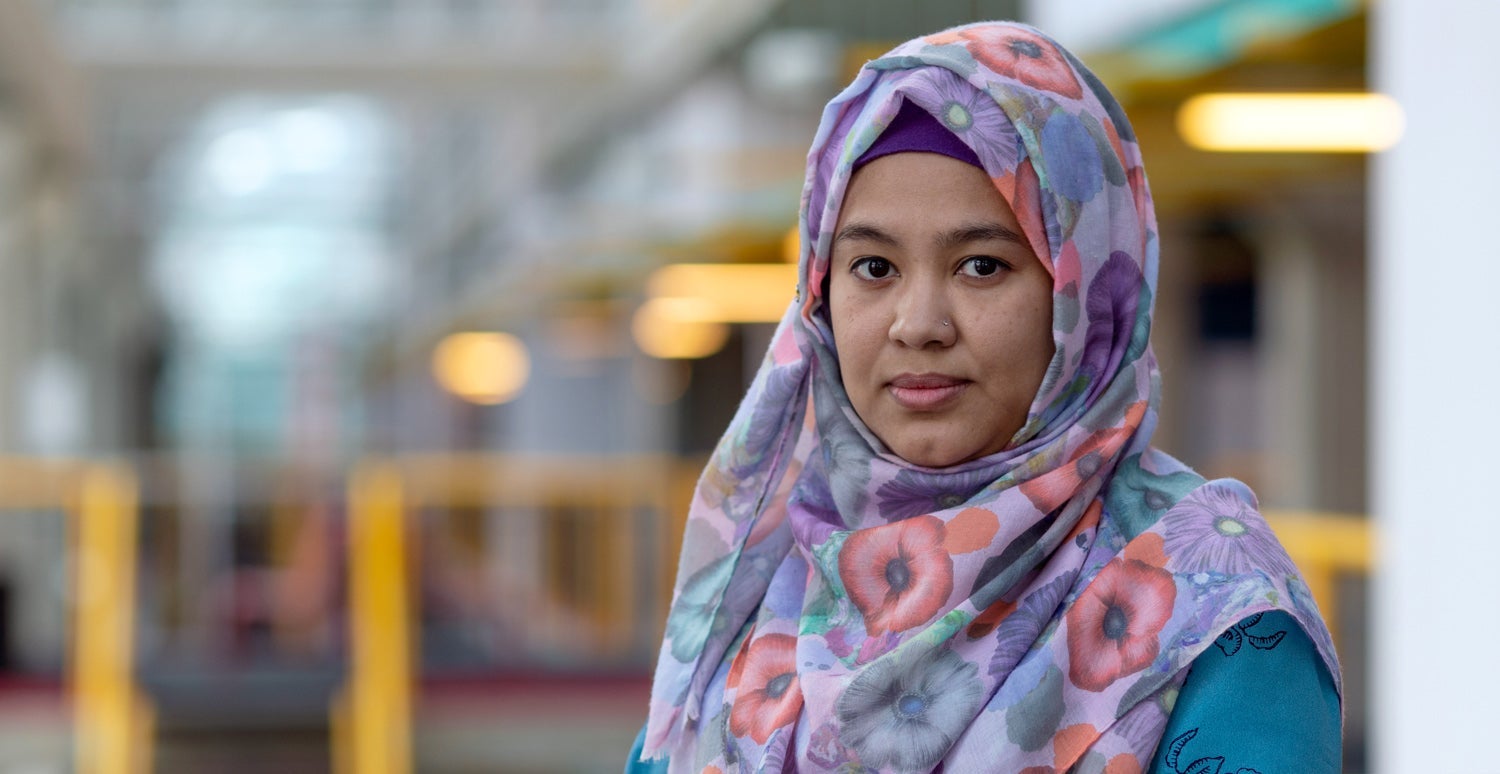
Fatema
Tuz
Zohora
is
a
PhD
student
working
in
the
Bioinformatics
research
group,
under
the
supervision
of
Ming
Li,
University
Professor
at
the
Cheriton
School
of
Computer
Science
and
the
Canada
Research
Chair
in
Bioinformatics.
Her
research
area
involves
the
application
of
deep
neural
networks
and
other
machine
learning
algorithms
in
proteomics
data,
as
well
as different
clinical
data,
to
develop
models
for
disease
biomarker
discovery.
She
is
also
an
Assistant
Professor
(on
study
leave)
in
the
Department
of
Computer
Science
and
Engineering
at
the Bangladesh
University
of
Engineering
and
Technology,
one
of
the
leading
universities
in
Bangladesh
for
studying
computer
science.
Peptides are the chains of amino acids that make up proteins in human tissue. It is these small chains that often display the specific markers of disease. Having better testing means it will be possible to detect diseases earlier and with greater accuracy.
Fatema’s team calls their new deep learning network PointIso. It is a form of machine learning that was trained on an enormous database of existing sequences from bio-samples.
“Other methods for disease biomarker detections usually have lots of parameters, which have to be manually set by field experts,” Fatema said. “But our deep neural network learns the parameters itself, which is more accurate, and makes the disease biomarker discovery approach automated.”
The new program is also unique in that it is not trained to only look for one kind of disease, but also to identify the biomarkers associated with a range of diseases, including heart disease, cancer and even COVID-19.
“It’s applicable for any kind of disease biomarker discovery,” Fatema continued. “And because it is essentially a pattern-recognition model, it can be used for detection of any small objects within a large amount of data. There are so many applications for medicine and science, it’s exciting to see the possibilities opening up through this research and how it can help people.”
To learn more about this research, please see Fatema Tuz Zohora, M. Ziaur Rahman, Ngoc Hieu Tran, Lei Xin, Baozhen Shan, Ming Li. Deep neural network for detecting arbitrary precision peptide features through attention based segmentation. Scientific Reports 11, 18249 (2021). https://doi.org/10.1038/s41598-021-97669-7.