PhD candidate Shenghao Yang is one of ten recipients nationally to receive a Borealis AI 2020–2021 Fellowship. Now in its fourth year, these $10,000 fellowships are conferred annually to exceptional students pursuing graduate studies in machine learning and artificial intelligence at universities across Canada.
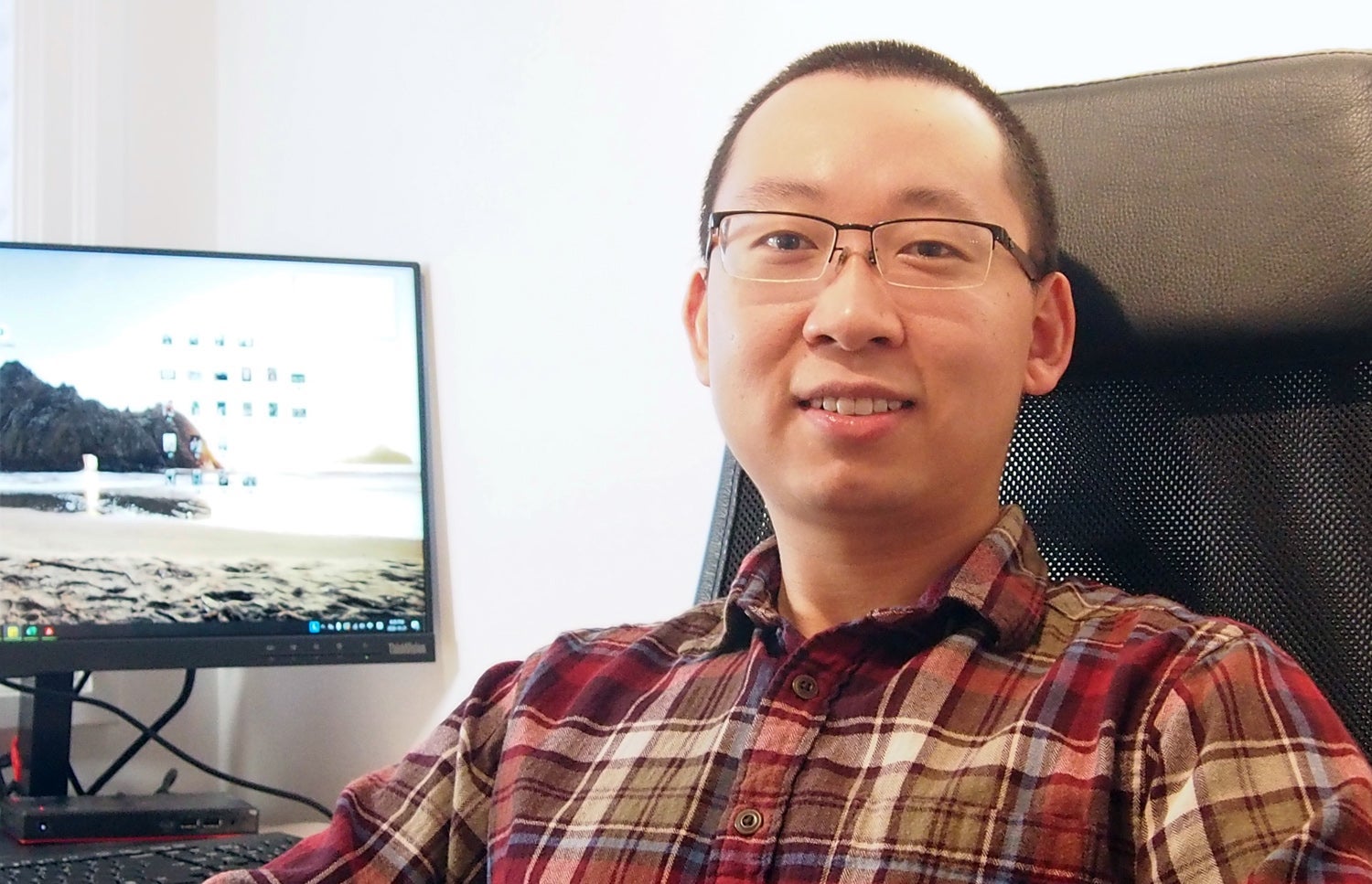
Shenghao Yang is a first-year PhD student in the Cheriton School of Computer Science, advised by Professor Kimon Fountoulakis. He is a member of OpAL lab and works on optimization and machine learning problems on graphs.
“Shenghao is an outstanding student and unquestionably deserving of a Borealis AI Fellowship,” said Cheriton School of Computer Science Professor Kimon Fountoulakis, who supervises Shenghao.
“During his undergraduate degree at Western University, Shenghao received two gold medals for highest academic average. He then came to Waterloo to pursue graduate studies in combinatorics and optimization, where he received the Sinclair Graduate Scholarship and the William Tutte Postgraduate Scholarship. He is now pursuing a PhD in computer science in my group and is showing clear signs that he will become a leader in algorithms and formulations for local graph clustering.”
About Shenghao Yang
Shenghao Yang joined Professor Fountoulakis’s research group as a master’s student in September 2019. His research was of such depth and calibre that shortly afterwards he switched to the PhD program. Within six months, Shenghao had published a paper titled p-norm flow diffusion for local graph clustering at ICML 2020, the 37th International Conference on Machine Learning, one of the top two conferences in the field. This paper combined the advantage of both continuous and combinatorial approaches to local graph clustering by formulating a class of local combinatorial diffusion processes as a family of convex optimization problems.
Shenghao also co-authored Parallel and communication avoiding least angle regression, a paper that was published recently in SIAM Journal on Scientific Computing. In this work, he implemented algorithms on distributed computers and performed extensive experiments to demonstrate the performance of the proposed methods.
Shenghao is also a coauthor of a timely paper titled Targeted pandemic containment through identifying local contact network bottlenecks, which is under minor revision for publication at PLOS Computational Biology. During the COVID-19 pandemic, politicians and public health officials have had to make difficult decisions about where to impose a lockdown and whom to quarantine. In this study, Shenghao and his coauthors examined a computationally efficient network centrality measure that enables detection of local transmission bottlenecks — contact edges in a group that are especially important for the spread of disease among small communities or local network structures inside large networks. This important paper shows that pandemic intervention strategies that target local network structures are significantly more effective than those that focus solely on the network structure as a whole, the strategy often thought to be the most effective way to limit the spread of COVID-19 in a community.
Borealis AI is a world-class AI research centre created by RBC, Canada’s largest financial institution. Established in 2016, with labs in Waterloo, Toronto, Montreal and Vancouver, Borealis AI supports open academic collaborations and works with world-class research centres in artificial intelligence. Recognized for scientific excellence, Borealis AI’s dedicated team of researchers and engineers builds AI-enabled products using state-of-the-art machine learning. Its research areas are diverse and include natural language processing, reinforcement learning and responsible AI.