Cheriton School of Computer Science Professors Sergey Gorbunov, Gautam Kamath and Jian Zhao have each been awarded a 2020 Natural Sciences and Engineering Research Council Discovery Accelerator Supplement.
“Congratulations to Sergey, Gautam and Jian for being awarded NSERC Discovery Accelerator Supplements,” said Professor Raouf Boutaba, Director of the David R. Cheriton School of Computer Science. “All three are exceptional young computer scientists working at the forefront of highly relevant and transformative areas in computer science. Their outstanding research programs span, respectively, cryptographic systems and protocols, statistical data science, and information visualization combining techniques from human-computer interaction and data science.”
NSERC Discovery Accelerator Supplements provide substantial and timely additional resources to accelerate progress and maximize the impact of established, superior research programs. Each supplement is valued at $120,000 and awarded over three years.
Professor Sergey Gorbunov
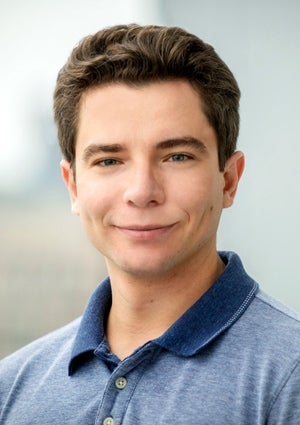
Research project: Enabling New Applications via Cryptographic Tools for Data In-Use
Background: Cryptography is a foundational science that allows the design of tools, protocols, and systems that protect everything from communications and data to programs and financial transactions. Classical cryptographic mechanisms served us well to transmit data securely from one user to another even when an adversary compromises the communication medium between the users. However, in recent years, we are seeing a fundamental shift in global computing.
Today, people use lightweight mobile devices, laptops, and IoT devices. These devices feed data from remote agents — for example, cloud providers and public ledgers — and query these agents to perform computations over the data. But can these models truly scale up to their potential while ensuring data and computation privacy? And could we allow for new types of applications if trust and privacy features were enabled by default into these environments?
What this research addresses: Sergey’s research will develop new theoretical models, constructions, and systems that enable new applications by protecting data in use and computations on untrusted agents. His research will study how to enable new types of applications that require privacy and security by default and will empower users to gain control of their personal data. Specifically, he and his students will design new security models, methods and algorithms for secure computation over encrypted data; construct new algorithms for secure processing of machine learning, artificial intelligence and natural language processing applications; and build systems and new applications that enable secure data and program processing in untrusted environments.
Professor Gautam Kamath
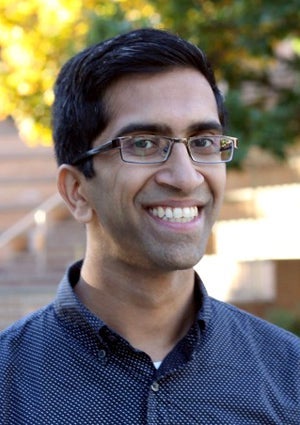
Research project: Theoretical Foundations of Differentially Private Statistics
Background: Given the ubiquity of large data sets, statistics and machine learning are used in many application areas to perform inference and prediction. However, many data sets contain sensitive personal information, so it is vital to ensure that the results of these procedures do not reveal private information. As an example, suppose researchers were conducting an analysis on genetic data from individuals who are HIV positive.
Recent results have shown that, under certain conditions, it is possible to reidentify individuals who participated in such a study. Given the social stigma associated with HIV/AIDS, reidentification would not only be a gross violation of individual privacy but could also discourage individuals from participating in research studies.
What this research addresses: The goal of Gautam’s research is to develop methods and tools for private statistics and, for a given task, answer the following central question: how much more data do we need to ensure that our solution to the task does not violate the privacy of the users?
Gautam’s work advances both theory and practice. First, he and his group will develop new algorithms and analyses to solve more complex and general tasks. Second, they will study privacy settings that match those applied in practice at large-scale deployments, and design time and data efficient algorithms for those settings. Last, he will experiment with and tune theoretical algorithms to make code that is effective on real data.
While significant work has been conducted on privacy and statistics, this work differs in two important ways — specifically, a focus on understanding properties of the underlying population, rather than on a specific data set, and investigating the cost of privacy with finite amounts of data. As statistical methods are becoming only more and more common, and privacy concerns are an increasingly common topic of public discourse, the importance of rigorous methods for private statistics is paramount. In particular, because of recent events demonstrating the power of massive amounts of user data — such as the Facebook–Cambridge Analytica data scandal — significant amounts of new policy are likely to be implemented to prevent such events from reoccurring. In turn, this will necessitate training highly qualified personnel in data privacy at companies that deal with user data — roles that Gautam’s graduate students will fill.
Professor Jian Zhao
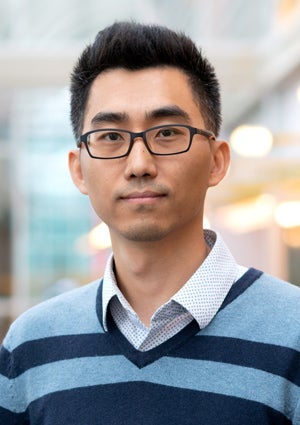
Jian’s research focuses on information visualization, human-computer interaction, and data science. He develops advanced interaction and visualization techniques that promote the interplay between humans, machines, and data.
Research project: Visualization Techniques for Collaborative Data Analysis
Background: We are continuously generating large amounts of data in different forms, and facing more complicated problems related to the data. With recent technological advances in big data analytics, many well-defined questions can be automatically solved by machines. However, many data problems are still ill-defined, vague, and exploratory, and they require close human engagement and supervision.
Information visualization and human-computer interaction are powerful techniques to help people understand abstract data and algorithms with visual representations and user interactions. However, because of the scale and complexity of the data problems, multiple analysts with diverse backgrounds need to work together. Furthermore, of the three key players — data, machines, and humans — people at the centre has become a shared view for the approach to solve real-world problems. Humans undoubtedly need to be involved, but our potential for processing information is limited.
What this research addresses: Jian’s research aims to investigate visualization techniques for collaborative data analysis, by promoting the interplay between data, machines, and humans, as an overarching goal. He will pursue three interrelated objectives. The first is to develop visual analysis tools to provide insights into the collaborative behaviour and patterns of analysts. The second is to develop interactive visualization to support communication of abstract data and models in collaborative data analysis. The third is to design algorithms and visual metaphors to improve quality and efficiency of knowledge discovery in collaboration.
This research will result in new visualization techniques, systems and methods that allow us to better understand human behaviour during collaboration and improve the efficiency of analysts in collaborative data analysis. The outcomes will form an infrastructure or repository to be reused for broader interests in immediate domains such as data science as well as in other fields such as social science.