Professor Yaoliang Yu has been awarded $100,000 by the Ministry of Colleges and Universities Early Researcher Awards program to develop deep generative machine learning models. The Ministry’s amount is matched by an additional $50,000 from the University of Waterloo, bringing the total funding to $150,000 to support one PhD, two master’s, and a number of undergraduate students over five years.
Professor Yu is one of five researchers at Waterloo to receive a 2024 Early Researcher Award, a provincial program that recognizes exceptional young faculty by helping them expand their research team.
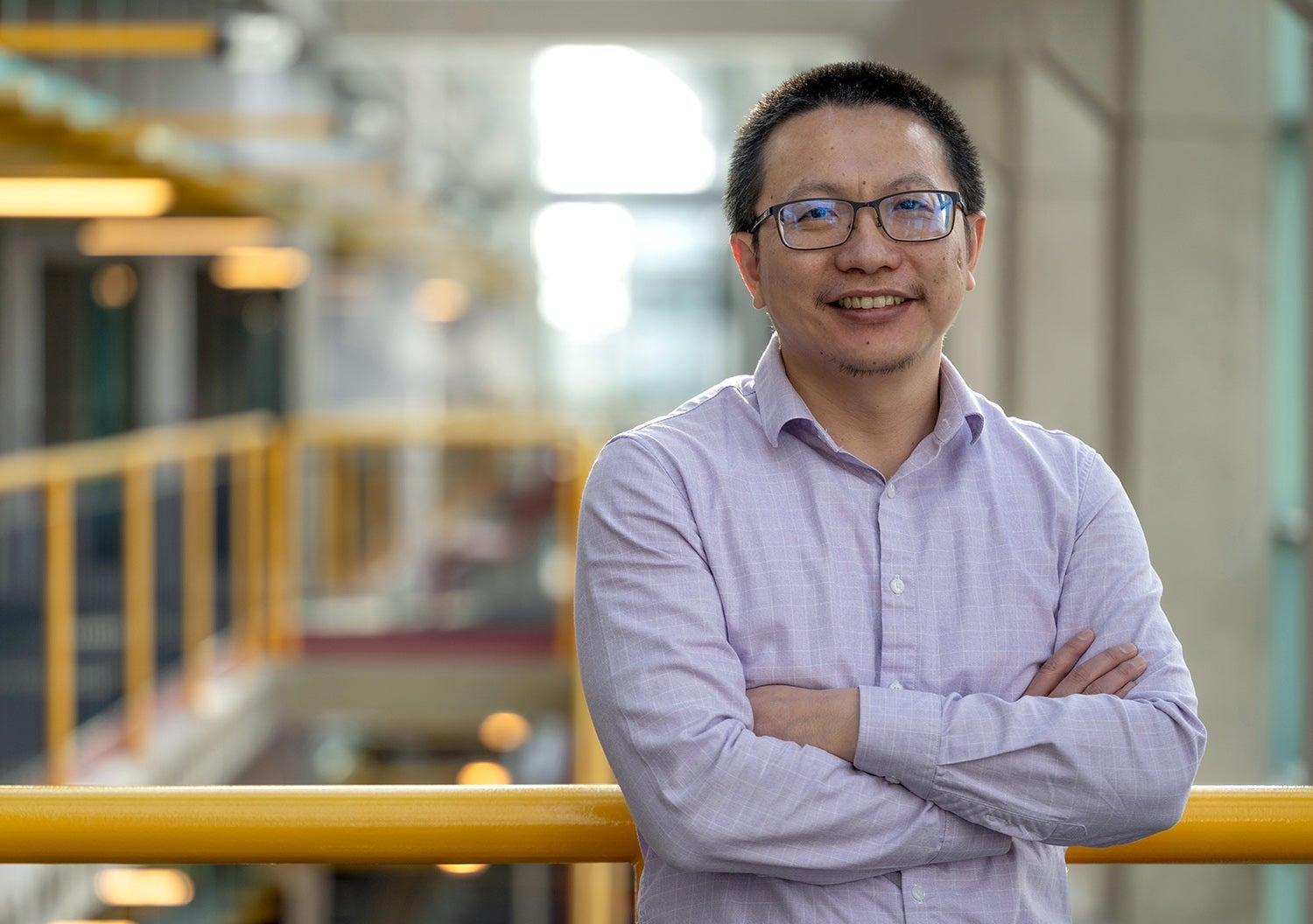
Professor
Yaoliang
Yu
is
an
expert
in
machine
learning
and
optimization.
He
is
a
faculty
member
at
the
Vector
Institute,
a
Canada
CIFAR
AI
Chair,
and
was
a
Cheriton
Faculty
Fellow
from
2020
to
2023.
He
has
won
best
paper
awards
at
ACM
SIGSOFT
as
well
as
at
multiple
workshops,
and
has
published
more
than
70
top
journal
and
conference
papers
in
machine
learning.
His
research
has
been
cited
more
than
4,000
times
with
an
h-index
of
31
as
of
March
2024
according
to
Google
Scholar.
Over
the
past
decade,
he
has
supervised
and
co-supervised
nine
undergraduate,
six
master’s
and
ten
doctoral
students.
“We are immensely grateful to the Ontario government for their investment in the University of Waterloo’s researchers through the Early Researcher Awards,” says Charmaine Dean, vice-president, Research and International. “Waterloo is advancing innovation in many impactful areas to develop new technologies and boost economic development.”
Background
After years of foundational research, we are witnessing a truly explosive growth of artificial intelligence. Almost monthly another significant breakthrough is heralded, from AlphaGo, the computer program that beat the best human players at Go, to AI agents that have set new records in determining a protein’s 3D shape based on the sequence of its constituent amino acids, to restoring and determining provenance of ancient texts, to solving open problems in math and computer science, among many more developments.
Among the factors that have contributed to AI’s success is its ability to process huge amounts of data using unsupervised learning, a type of machine learning that does not require manual labelling of data or reinforcement by humans. Perhaps the simplest way to achieve unsupervised learning is by using generative modelling. The well-founded assumption is that if the models can automatically generate data they must have gained significant knowledge and are ready to generalize.
But as impressive as they are, deep generative models are difficult and expensive to train because they require much data and computing power. In fact, training has become so expensive that often it is not possible to fix a bug discovered after a model has been trained. Moreover, as training involves more and more user data and computing power, containing costs and striking a balance between model utility, robustness against malicious use, and privacy all become increasingly important.
About Professor Yu’s proposed research
With support from the Early Researcher Awards program, Professor Yu and his students will address these issues by creating lightweight generative models that require significantly less data, storage and computing power, by developing distributed systems that enable training on low-cost smart devices, and by accounting explicitly for concerns about trustworthiness.
Generative models are an indispensable part of unsupervised learning and artificial intelligence. In short, a generative model learns to generate new data similar to data it has been given. A new emerging theory formulates generative models as learning a sequence of deterministic mappings that turn input data — e.g., a photograph of a dog — gradually into noise. By reverting this process new data can be generated from pure noise.
The reverse map — termed a push-forward in probability theory — can be parameterized by deep neural networks whose weights are then learned through stochastic gradient algorithms. This new method has been extremely fruitful and has revealed surprising connections between different algorithms and fields. Professor Yu’s proposed research aims to develop this idea of push-forward substantially and to broaden the horizon of generative modelling significantly.