Muhammad Sulaiman, Mahdieh Ahmadi, Mohammad A. Salahuddin, and Raouf Boutaba from the Cheriton School of Computer Science and their colleague Aladdin Saleh from Rogers Communications have won the NOMS 2023 Best Paper Award for “Generalizable Resource Scaling of 5G Slices using Constrained Reinforcement Learning.”
Notably, this achievement follows the research group’s previous recognition at NOMS 2022, where they were honoured with the Best Paper Award as well.
Their award-winning research was presented at the 36th IEEE/FIP Network Operations and Management Symposium, which this year explored the theme of integrated management towards resilient networks and services.
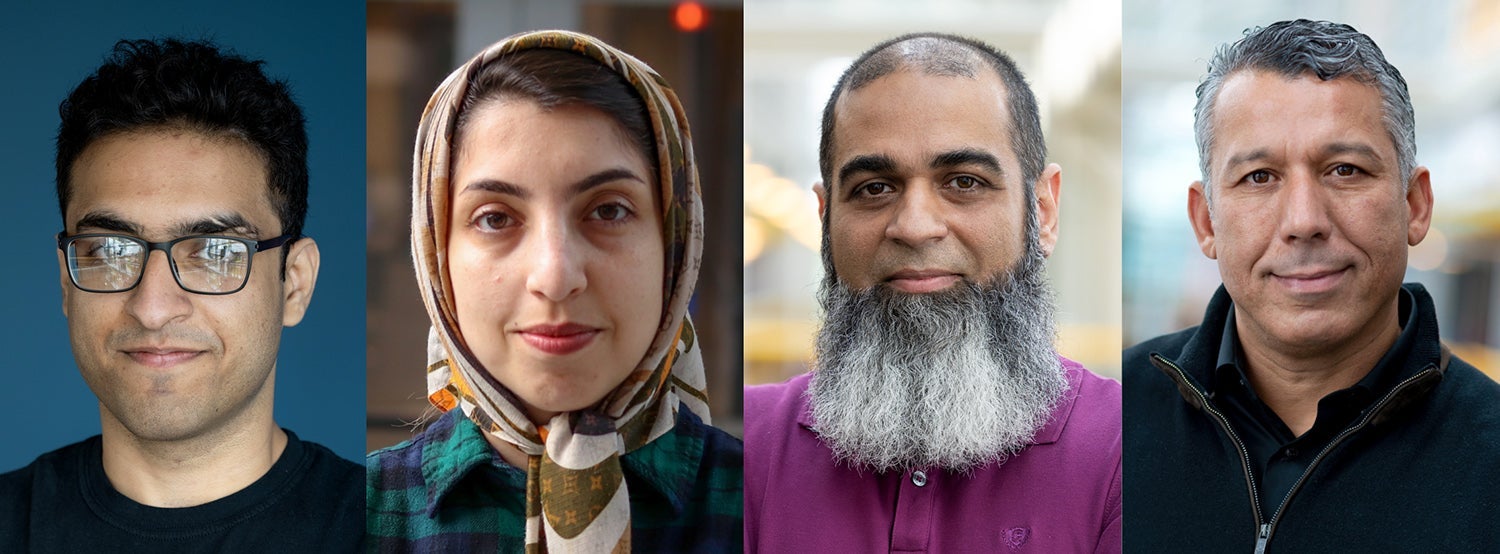
L to R: PhD candidate Muhammad Sulaiman, Postdoctoral Researcher Mahdieh Ahmadi, Research Professor Mohammad A. Salahuddin, and Professor Raouf Boutaba. Aladdin Saleh’s photo was unavailable.
Background
5G mobile networks are moving away from a one-size-fits-all approach to networking to one where a network’s architecture is programmable. By using software-defined networking and by virtualizing the network functions, an infrastructure provider can create virtual isolated networks — known as network slices — over a shared physical network infrastructure.
Network slicing allows 5G mobile networks to host applications and services with different quality-of-service requirements. For example, 4K video streaming can use network slices that provide high throughput with lenient latency, as the key requirement of ultra-high definition video is a high data rate. Other applications such as telesurgery, a type of surgical procedure performed when a surgeon and patient are in different locations, requires ultra-reliable, low-latency network slices, because here consistent and timely feedback is critically important to a surgeon conducting a procedure remotely.
When a service provider requests a network slice from an infrastructure provider it includes both the peak traffic and the minimum quality of service required in the service level agreement. The required resources to maintain a slice’s quality of service depend on its type and traffic, which varies with time. The infrastructure provider can guarantee the quality of service by allocating isolated resources to the slice based on its peak traffic, but doing so can lead to over-provisioning because the actual traffic of a slice rarely reaches its peak.
To improve resource efficiency, an infrastructure provider can predict future traffic on a slice and scale its resources accordingly. However, under-provisioning the resources, whether due to inaccurate traffic prediction or imprecise network modelling reduces the quality of service of the slice. For this reason, a certain level of quality-of-service degradation is usually incorporated into service level agreements, and the goal of infrastructure providers is to scale resources dynamically to maximize resource efficiency while keeping the quality of service degradation under the specified limit.
This is known as dynamic resource scaling, and several challenges need to be addressed to effectively achieve it. Machine learning solutions require a dataset to be trained, but the traffic that a slice experiences may be unknown during training. Given the uncertainty of network conditions and future traffic, and the complex modelling of the end-to-end network, designing an algorithm that can scale the resources of the slices dynamically while keeping their quality-of-service degradation below the agreed-upon threshold is challenging.
Research contributions
The research team used a regression-based model to capture the behaviour of an end-to-end network operating under different conditions. Their model was trained offline using a dataset gathered by measuring the performance of an isolated slice in a real network under diverse network conditions and with different amounts of allocated resources.
Regression-based models, such as neural networks, can learn complex relationships without requiring an exact mathematical model. To scale the resources allocated to the slice dynamically while satisfying quality-of-service requirements, the team used constrained deep reinforcement learning with offline training. Although offline training addresses the slow training problem of online training, it must be generalizable to online traffic patterns not seen during offline training. For this purpose, they used a risk-constrained deep reinforcement learning algorithm coupled with domain randomization.
Risk-constrained deep reinforcement learning increases the chances of meeting quality-of-service degradation constraints under unpredictable traffic and network conditions by constraining the percentile-risk rather than just the expected value of quality-of-service degradation. Domain randomization, on the other hand, is a common technique to bridge the simulation-to-reality gap by randomizing the environment parameters during training. Additionally, the reinforcement learning agent is fed the output of an external traffic prediction module to avoid overfitting to any specific traffic pattern.
The main contributions of their research are the following:
- Developing a novel framework for dynamic resource scaling that consists of a regression-based network model, a risk-constrained deep reinforcement learning agent, and a traffic prediction module. By training the risk-constrained reinforcement learning agent offline using random traffic, they devised a generalizable agent that did not require prior knowledge of online slice traffic patterns.
- Evaluating the effectiveness of the proposed approach against traditional and constrained deep reinforcement learning-based models. In general, their approach performed relatively better than others while also showing generalization to previously unseen traffic and network conditions.
- Assessing the robustness of the proposed approach under varying network conditions and inaccurate traffic predictions and demonstrating that it can effectively scale resources even under worse-case scenarios.
- Demonstrating that the pre-trained model can be fine-tuned to further increase performance while meeting quality-of-service degradation constraints and maintaining generalizability.
Current state-of-the-art approaches can lead to quality-of-service degradation as high as 44.5% when tested on previously unseen traffic. In contrast, the research team’s approach maintains quality-of-service degradation below any pre-set threshold on such traffic, while minimizing the allocated resources. Additionally, they demonstrated that the proposed approach is robust against varying network conditions and inaccurate traffic predictions. Their next steps are to extend the evaluations to multiple types of resources and slices and to validate their approach on an expansive 5G testbed.