Cheriton School of Computer Science Professor Shai Ben-David, his former PhD student Hassan Ashtiani, now an Assistant Professor at McMaster University, along with colleagues Christopher Liaw, Abbas Mehrabian and Yaniv Plan, have received a best paper award at NeurIPS 2018, the 32ndAnnual Conference on Neural Information Processing Systems.
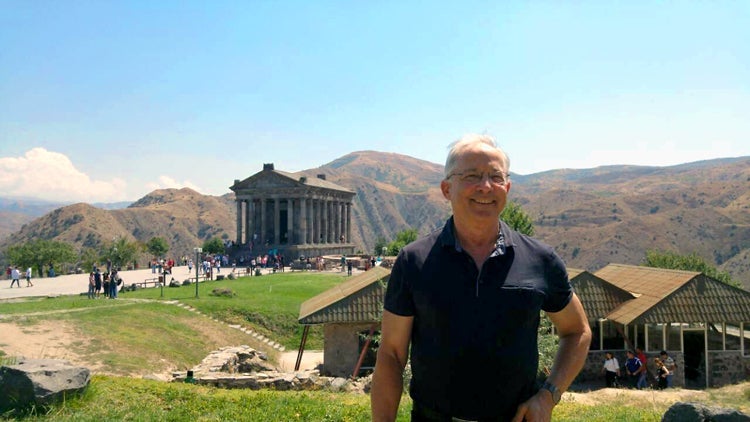
Professor Shai Ben David
NeurIPS (formerly NIPS) is the largest and most important annual conference for machine learning. It attracts more than 5,000 paper submissions and many thousands of participants. This year, the conference was held from December 3 to 8, 2018 in Palais des congrès de Montréal, a large convention centre in Montréal.
Their paper is titled Nearly tight sample complexity bounds for learning mixtures of Gaussians via sample compression schemes. Estimating distributions from observed data is a fundamental task in statistics that has been studied for more than a century. This task also arises in applied machine learning and it is common to assume that the distribution can be modelled using a mixture of Gaussians.
“The sample complexity of distribution learning problems is the number of samples you need to have a guarantee that you’re close to the true model that generates the data,” explains Professor Ben-David. “We figured out the exact sample complexity for the important family of mixtures of Gaussians in Euclidean spaces with almost matching lower bound and upper bounds.”
“Congratulations to Shai, Hassan and their colleagues at the University of British Columbia,” said Dan Brown, Director of the David R. Cheriton School of Computer Science.
“Shai and Hassan were working on minimizing the number of examples to model using a mixture of Gaussians while another research team at UBC was doing related work — a team that includes Nick Harvey, formerly an Assistant Professor in the Department of Combinatorics and Optimization, and Abbas Mehrabian, a postdoctoral fellow at McGill who was awarded a Governor General’s Gold Medal for high standing academic achievement for his PhD while in the Department of Combinatorics and Optimization. The team pooled their efforts to prepare this exemplary joint work for which they have received a best paper award.”
To learn more about this research, please see Hassan Ashtiani, Shai Ben-David, Nick Harvey, Christopher Liaw, Abbas Mehrabian, Yaniv Plan. Nearly tight sample complexity bounds for learning mixtures of Gaussians via sample compression schemes. 2018, 32ndConference on Neural Information Processing Systems (NeurIPS 2018), Montréal, Canada.