The University of Waterloo has recognized recent PhD graduate Dr. Nils Lukas with the 2024 Alumni Gold Medal for his exceptional academic performance in a doctoral program. This prestigious award, conferred by Alumni Relations, features a custom-crafted, 24-carat gold-plated medal embossed with the university’s seal. Since 1970, the Alumni Gold Medal has been conferred to top graduating students from Waterloo’s six faculties at convocation.
“It is exciting to receive the 2024 Alumni Gold Medal and I am honoured to have been selected,” said Dr. Lukas, now an Assistant Professor at the Mohamed bin Zayed University of Artificial Intelligence (MBZUAI) in Abu Dhabi. “I owe immense gratitude to my advisor, Florian Kerschbaum, for his exceptional mentorship. I look forward to continuing my research on secure and private machine learning at MBZUAI.”
Dr. Lukas’s research focuses on some of the most pressing security and privacy challenges in machine learning, including issues related to untrustworthy data, providers, and users, explained Professor Kerschbaum.
“It has been a great pleasure to work with Nils,” Professor Kerschbaum said. “He is a truly insightful researcher, whose work is outstanding not only in quantity but more importantly in quality, as his insights always bring a fresh perspective to real-world problems.”
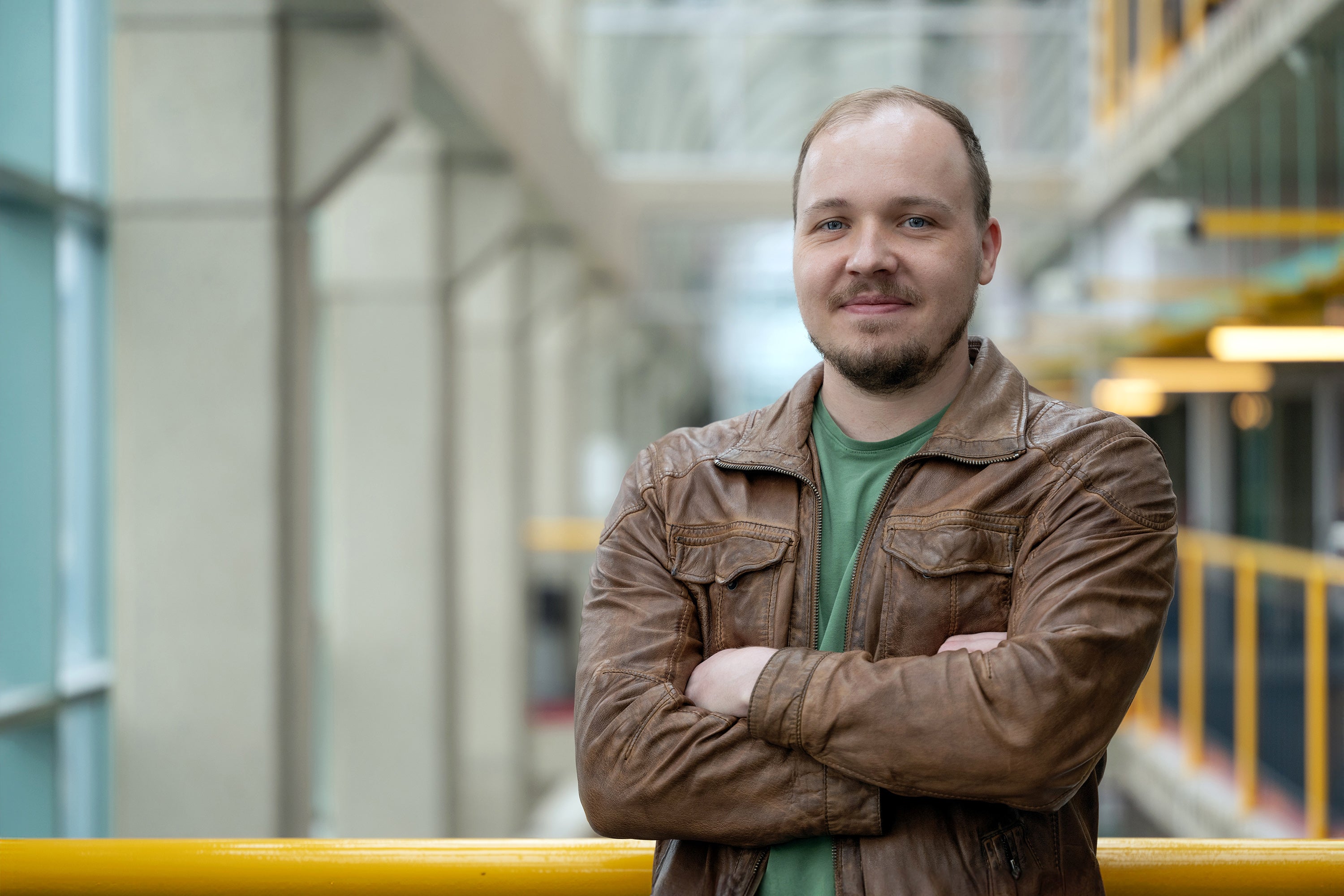
Nils Lukas is an Assistant Professor at MBZUAI in Abu Dhabi. His research focuses on designing secure and private machine learning systems in the presence of untrustworthy providers, data, models and users. He earned his PhD at the University of Waterloo and his MSc with distinction in Computer Science from RWTH Aachen University in Germany.
His research explores the threats that arise when deploying deep neural networks from three perspectives: (1) privacy when the model is trained on private data, (2) reliability when the model’s training data cannot be trusted, and (3) model misuse when the users cannot be trusted. His work includes studying privacy attacks against large language models fine-tuned on private datasets, developing defences against data poisoning, and creating multiple methods for controlling model misuse.
Among his recognitions at Waterloo, Dr. Lukas was a first-place winner of the 2024 Faculty of Mathematics Doctoral Prize. He held a prestigious 2022–24 Cheriton Graduate Scholarship, and his research has won two notable poster competitions: the 2023 Cheriton Research Symposium poster competition and the 2019 Cybersecurity and Privacy Institute poster competition.
More about Nils Lukas’s research
The rapid advancement of generative AI models in recent years holds great promise to transform businesses and society, but they also pose novel trust, security and privacy challenges. Dr. Lukas’s research addresses these concerns, particularly in the context of machine learning models.
In his 2023 paper titled Analyzing Leakage of Personally Identifiable Information in Language Models, published in IEEE Symposium on Security & Privacy with colleagues from Microsoft Research, Dr. Lukas introduced novel attack algorithms capable of extracting ten times more personally identifiable information than existing attacks. This work revealed that standard sentence-level differentially private training, while largely reducing the risk of disclosing personally identifiable information, still leaks about 3% of such information. The significance of this work is that it is one of the first comprehensive studies of the risk of personally identifiable information memorization in language models, and it exposed the subtle insufficiency of sentence-level differentially private training for protecting record level personally identifiable information. Dr. Lukas has released his code to the public to reproduce and conduct further research.
Another significant contribution came from his 2022 paper, SoK: How Robust is Image Classification Deep Neural Network Watermarking?, research with Edward Jiang, Xinda Li and Florian Kerschbaum presented at IEEE Symposium on Security & Privacy. In this work, Dr. Lukas conducted a systematic evaluation of the robustness of existing watermarking schemes that aim to verify provenance of machine learning models and to prevent misuse of AI generated content. They found that none of the surveyed watermarking schemes can withstand all removal attacks, showcasing the importance of a thorough evaluation framework.
In Deep Neural Network Fingerprinting by Conferrable Adversarial Examples, a paper with Yuxuan Zhang and Florian Kerschbaum presented at ICLR 2021, Dr. Lukas developed a fingerprinting method for deep neural networks, aimed at detecting the surrogate models that an adversary may build by querying a proprietary source model. The team proposed a new method to generate conferrable adversarial examples and, importantly, demonstrated their superior effectiveness and robustness against previous fingerprints and watermarks.
In PTW: Pivotal Tuning Watermarking for Pre-Trained Image Generators, a paper with Florian Kerschbaum presented at the 32nd USENIX Security Symposium, Dr. Lukas explored image generators, such as those used in deepfake creation. He proposed pivotal tuning watermarking to prevent misuse of image generators, achieving three orders of magnitude speedup while obviating the need of any training data. Moreover, he revealed some intrinsic trade-off between the undetectability and robustness of watermarks.
In Leveraging Optimization for Adaptive Attacks on Image Watermarks, a paper with Abdulrahman Diaa, Lucas Fenaux, and Florian Kerschbaum presented at ICLR 2024, the authors continued the investigation of image watermarking attacks through the lens of adaptive, learnable attacks. The core idea is that an adaptive attacker who knows the watermarking algorithm can create their own surrogate keys and use them to optimize the parameters of a watermark removal attack. Such adaptive, learnable attacks can undermine the robustness of all five tested, state-of-the-art watermarking methods and require limited computational resources. Dr. Lukas has presented his watermarking results to Google, with the goal that the research will limit misuse of its image generators and combat misinformation.