Akshay Ramachandran has received two awards for his doctoral research — the 2022 Cheriton Distinguished Dissertation Award as well as second place in the 2022 Mathematics Doctoral Prize for his thesis titled “Geodesic convex analysis of group scaling for the Paulsen problem and tensor normal model.”
The Cheriton Distinguished Dissertation Award was established in 2019 to recognize excellence in computer science doctoral research at the School of Computer Science. In addition to the recognition, recipients of the award receive a cash prize of $1,000. The Mathematics Doctoral Prize, also conferred annually, recognizes the achievements of the top three graduated doctoral students in the Faculty of Mathematics. As a second-place winner of this award, Akshay will receive a $1,000 prize.
About Akshay Ramachandran and his research
Akshay was a PhD student in the Cheriton School of Computer Science’s Algorithms and Complexity group from August 2016 to October 2021, and advised by Professor Lap Chi Lau.
“Akshay’s thesis is a very significant piece of work,” said Professor Lau. “The problem is important, the result is optimal, the ideas are creative, the techniques are broad and deep, the final result is entirely on his own, and his dedication is phenomenal.”
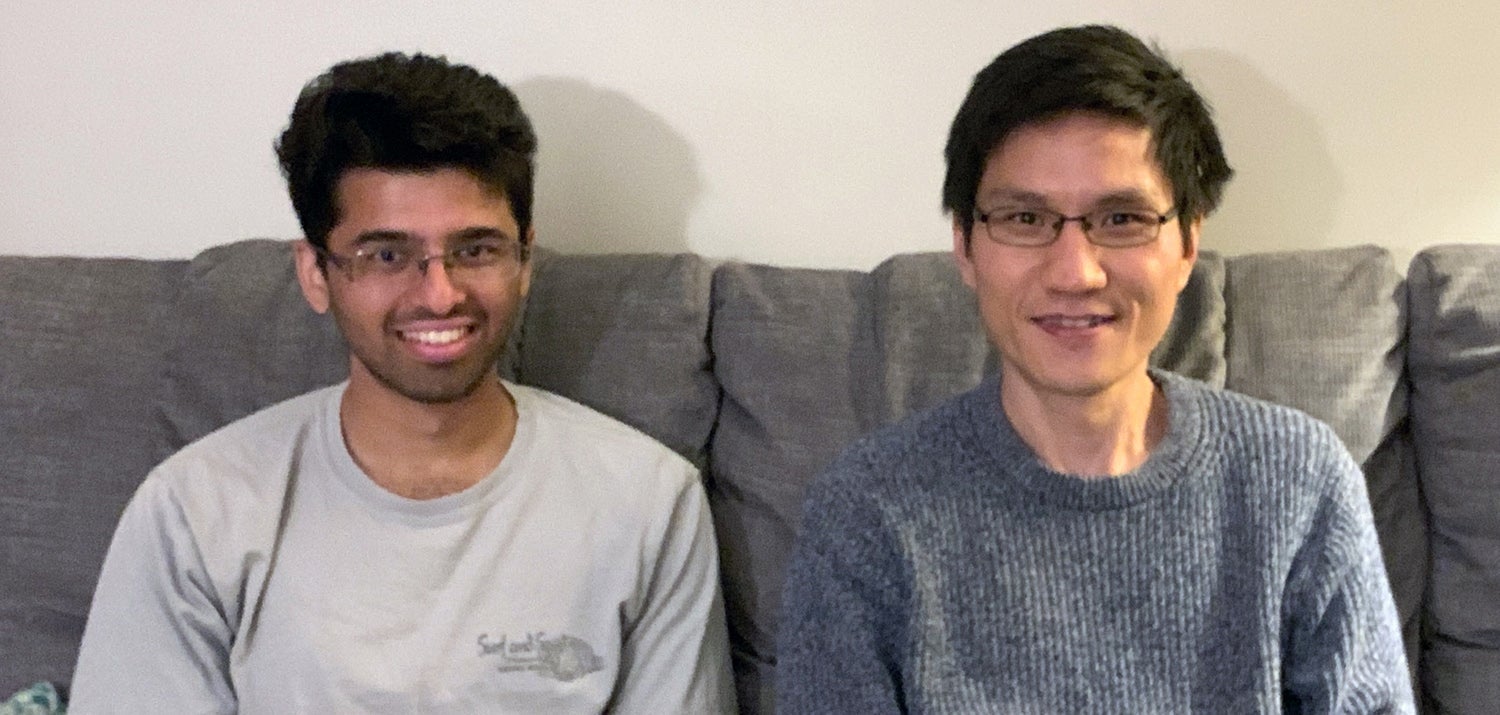
L
to
R:
Akshay
Ramachandran
and
his
PhD
advisor,
Cheriton
School
of
Computer
Science
Professor
Lap
Chi
Lau.
Akshay
is
currently
a
postdoctoral
researcher
at
the
University
of
Amsterdam
and
Centrum
Wiskunde
&
Informatica,
the
national
research
institute
for
mathematics
and
computer
science
in
the
Netherlands.
Akshay’s thesis presented two applications of matrix and tensor scaling methods. The framework of scaling problems has stimulated much interest in the theoretical computer science community because it has a variety of applications ranging from algebraic complexity to machine learning. Akshay first considered a basic open problem in frame theory known as the Paulsen problem, named after Pure Mathematics Professor Vern Paulsen, who coincidentally joined Waterloo’s Faculty of Mathematics around the time Akshay had begun his PhD studies.
The Paulsen problem, which had stymied mathematicians for two decades, asks whether any set of n vectors in d-dimension that forms an epsilon-nearly equal norm Parseval frame is close to an exact equal norm Parseval frame. The conjecture is that the distance between an epsilon-nearly equal norm Parseval frame and an exact equal norm Parseval frame is independent on the number of vectors n. This conjecture is the basis of a numerical approach to generate an equal norm Parseval frame, an important object in frame theory with various applications.
Akshay began working on this problem when he started his PhD studies. After more than a year of work, he, Professor Lau and their colleagues, Tsz Chiu Kwok at Waterloo and Yin Tat Lee at the University of Washington, proved that the distance is independent of the number of vectors n. The paper was accepted for presentation at STOC 2018, one of two top conferences in theoretical computer science. Further work on this problem resulted in an additional paper titled Spectral analysis of matrix scaling and operator scaling, which was accepted at FOCS 2019, the other top conference in theoretical computer science.
In the first part of his thesis, Akshay presented a principled framework, based on geodesic convexity, to improve and simplify these two results to obtain the optimal bound for the Paulsen problem. This is a deep result that required significant knowledge and Akshay’s years of effort.
In the second part of his thesis, Akshay considered the tensor normal model, research conducted jointly with his collaborators Professors Cole Franks at MIT, Rafael Oliveira at the University of Waterloo, and Michael Walter at Centrum Wiskunde & Informatica.
The problem they tackled is the following: if given a sample from a D-dimensional Gaussian whose covariance has a tensor structure, can the model be estimated by exploiting the tensor structure? Akshay and his colleagues were able to generalize their improved scaling results to higher-order tensors and give error bounds for the maximum likelihood estimator of the tensor normal model. Their sample complexity bounds are only a single dimension factor greater than necessary for the maximum likelihood estimator to exist at all. They also explained why the well-studied flip-flop algorithm performs well in practice by giving the first rigorous analysis of this algorithm, showing that it converges exponentially to the maximum likelihood estimator with high probability. This work resulted in a paper titled Near optimal sample complexity for matrix and tensor normal models via geodesic convexity.
Akshay is currently a postdoctoral researcher at the University of Amsterdam and Centrum Wiskunde & Informatica, where he collaborates with Professors Daniel Dadush and Michael Walter.