Master’s graduate Abbas Abou Daya, Research Professors Mohammad A. Salahuddin and Noura Limam, and Cheriton School of Computer Science Director Raouf Boutaba have received a CNOM best paper award for their research presented at the 16th IFIP/IEEE International Symposium on Integrated Network Management and published in the IEEE Transactions on Network and Service Management.
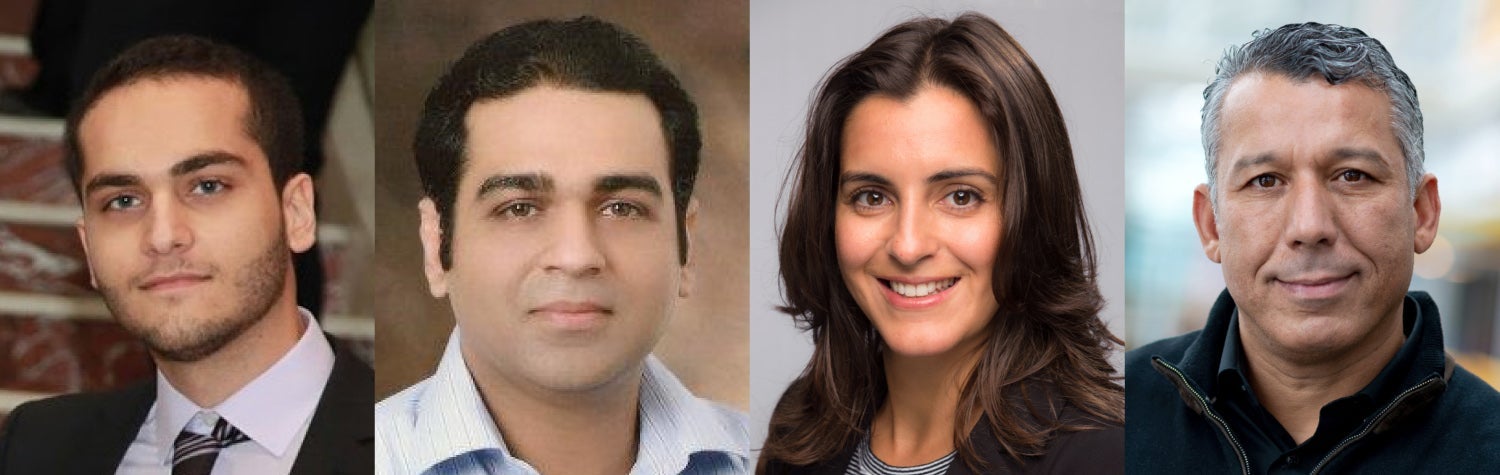
L to R: Master’s CS graduate Abbas Abou Daya, Research Professors Mohammad A. Salahuddin and Noura Limam, and Cheriton School of Computer Science Director and Professor Raouf Boutaba
Their award-winning paper, A Graph-Based Machine Learning Approach for Bot Detection, proposes a graph-based bot detection system that uses unsupervised and supervised machine learning to detect malicious agents in a network. Their contribution to cybersecurity is particularly relevant to both industry and individuals, as bots and botnets cause billions of dollars in losses annually across the globe.
A botnet is a number of Internet-connected devices, each of which is running one or more bots, that are controlled by botmasters through command and control channels. An adversary controls the bots through the botmaster, which can be distributed across several agents either within or outside a network. Bots can be used in a variety of malicious ways — from distributed denial-of-service attacks to massive-scale spamming to fraud and identity theft.
Detecting bots with classic network flow-level features using machine learning has been studied extensively, but approaches typically incur a high computational overhead, do not capture the network communication patterns, and can be easily subverted. Recently, bot-detection systems that leverage communication graph analysis using machine learning have helped overcome these limitations. A graph-based approach is particularly promising, as graphs represent the true communication behaviour of network hosts.
“Our team developed a two-phased, graph-based bot detection system called BotChase that leverages unsupervised and supervised machine learning,” said Professor Boutaba.
“The first phase prunes network hosts that are presumably benign, and the second detects bots with high precision. The prototype implementation, called BotChase, detects multiple types of bots and is robust to zero-day attacks — vulnerabilities that hackers exploit before software developers can develop a fix. Compared with other state-of-the-art approaches, BotChase outperforms end-to-end systems that employ flow-based features, it accommodates different network topologies, and it is suitable for large-scale data.”
To
learn
more
about
their
award-winning
paper,
please
see
Abbas
Abou
Daya,
Mohammad
A.
Salahuddin,
Noura
Limam,
Raouf
Boutaba.
BotChase:
Graph-based
Bot
Detection
Using
Machine
Learning,
IEEE
Transactions
on
Network
and
Service
Management,
vol.
17,
no.
1,
pp.
15–29,
March
2020.