My research is on data management. These days I would characterize it as data engineering aspects of data science. The main focus of my research follows two threads: (1) application of database technology to non-traditional data types, and (2) distributed & parallel data management. These two threads usually converge.
Investigating how database technology can be applied to data types that are more complex than business data processing (for which relational systems are the perfect fit) has always been one of my interests. Frank Tompa characterizes this type of work as Data Management(X) where X is the data type of interest -- we also have a graduate course with exactly this focus (CS 741). At different times in my career, X has been equal to one or more of the following: "object" data (in the sense of object databases), multimedia data, temporal data, spatial data, XML, stream data. Currently, my focus is on graph data and RDF data.
Graph data management
Graphs have always been important data types for database researchers. With the recent growth of social networks, Wikipedia, Linked Data, RDF, and other networks, the interest in managing very large graphs have again gained momentum. I have a number of projects in this space.
- A major focus of my current work in this area is high-speed streaming graphs. With PhD students Anil Pacaci and Aida Shehbolouki we are investigating query processing and analytics issues over these graphs. This work is part of the S-Graffito project which has its own page with more details. Anil's focus was on building a query processor for persistent, online queries over streaming graphs, while Aida's focus is on analysis and mining over such graphs. Within streaming graph query processing project, Kerem Akillioglu is investigating cardinality estimation for query optimization as part of his MMath research. For this part, we collaborate with Prof. Angela Bonifati (Lyon 1 University).
- With PhD student Khaled Ammar and Prof. Semih Salihoglu, we are investigating the efficient and effective use of differential dataflow computation in executing a number of workloads on dynamic graphs.
- With PhD student Zeynep Korkmaz and Prof. Khuzaima Daudjee, we are looking at efficient storage structures and cache management techniques for performance improvements of disk-based graph data management sytems.
- I have an interest in using hardware accellerators and heterogeneous architectures for speeding up graph processing. Right now, I am collaborating with Prof. Lei Zou (Peking University) and his students on this research.
Relevant Projects
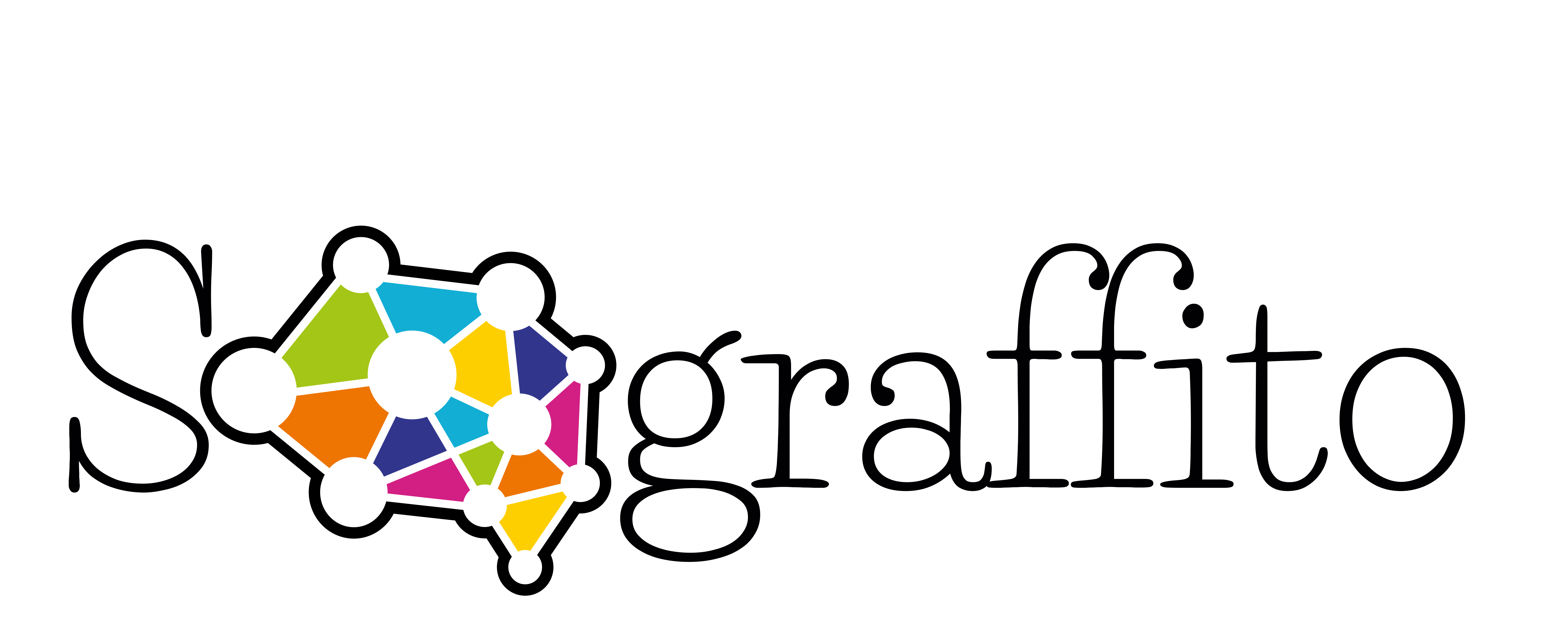
Publications
- A. Sheshbolouki and M. T. Özsu, sGrow: Explaining the Scale-Invariant Strength Assortativity of Streaming Butterflies, ACM Transactions on the Web, 2022. Accepted for publication.
- L. Zheng, L. Zou and M. T. Özsu. SGSI – A Scalable GPU-friendly Subgraph Isomorphism Algorithm. IEEE Trans. Knowledge and Data Eng., 2022. Accepted for publication
- A. Pacaci, A. Bonifati and M.T. Özsu, Evaluating Complex Queries on Streaming Graphs, In Proc. 28th IEEE Int. Conf. on Data Eng., pages 272-285, 2022.
- K. Ammar, S. Sadhu, S. Salihoglu and M. T. Özsu. Optimizing Differentially-Maintained Recursive Queries on Dynamic Graphs. Proc. VLDB Endowment, 15(11): 3186-3198, 2022.
- A. Sheshbolouki and M. T. Özsu. sGrapp: Butterfly Approximation in Streaming Graphs, ACM Transactions on Knowledge Discovery From Data, 16(4): Article 76, 2022.
- A. Pacaci, A. Bonifati and M. T. Özsu.Regular Path Query Evaluation on Streaming Graphs, In Proc. ACM SIGMOD International Conference on Management of Data, pages 1415-1430, 2020.
- L. Zeng, L. Zou, M. T.Özsu, L. Hu, and F. Zhang. GSI: GPU-friendly Subgraph Isomorphism. In Proc. 36th International Conference on Data Engineering, pages 1249-1260, 2020.
- A. Sahu, A. Mhedhbi, S. Salihoglu, J. Lin, M. T. Özsu.The Ubiquity of Large Graphs and Surprising Challenges of Graph Processing: Extended Survey, VLDB Journal, 29: 595–618, 2020.
- A. Pacaci and M. T. Özsu.Analysis of Streaming Algorithms for Graph Partitioning, In Proc. ACM SIGMOD International Conference on Management of Data, pages 1375–1392, 2019.
- X. Li and M. T. Özsu.Correlation Constraint Shortest Path over Large Multi-Correlation Graphs, Proc. VLDB Endowment, 12(5): 488-501, 2019.
- A. Sahu, A. Mhedhbi, S. Salihoglu, J. Lin, M. T. Özsu. The Ubiquity of Large Graphs and Surprising Challenges of Graph Processing, Proc. VLDB Endowment, 11(4): 420-431, 2018.
Other publications on this topic can be found here.
RDF data management
Resource Description Framework (RDF) has been proposed for modeling Web objects as part of developing the semantic web. It has also gained attention as a way to accomplish web data integration. For example, the Linking Open Data (LOD) cloud is a distributed RDF knowledge base created over hundreds of autonomous datasets. Currently, the LOD cloud contains more than 25 billion triples, and its size is doubling every year. As the volume of RDF data has increased, interesting data management issues have arisen. We study the processing of SPARQL queries over RDF data using a graph-theoretic approach: we represent both the RDF data and the SPARQL queries a graphs and conver the query evaluation problem toone of subgraph matching. My work in this area covers a number of topics:
- With Prof. Lei Zou (Peking University) and Prof. Lei Chen (Hong Kong University of Science and Technology) we investigated the development of a native RDF data management system called gStore that executes SPARQL queries using graph pattern matching techniques. gStore code base is maintained at Peking University and has been released as open source. We continue to work, now joined on this system by adding scalability through scale-out execution, efficiency through the use of modern hardware (GPU and FPGA) and other ways. See publications and gStore site.
- Prof. Lei Zou (Peking University), Prof. Peng Peng (Hunan University) and I collaborate on a number of topics related to distributed RDF processing, including data partitioning and efficient query processing.
- Former PhD student Güneş Aluç's research focused on the adaptive management of RDF data. Although some existing techniques address adaptivity to changes in the RDF data, they do not consider adaptivity to the changes in the workload. Consequently, the underlying database layouts and the indexes created over the databases are fixed a priori, and they cannot easily handle changing workloads. In fact, our experiments demonstrate that existing systems are unable to achieve consistently good performance across different types of SPARQL workloads, and that there can be orders of magnitude difference between the peak and base performance of a system.
- Güneş Aluç led, with the participation of former postdoc Olaf Hartig and Prof. Khuzaima Daudjee, the development of an RDF system benchmark called WatDiv to measure how an RDF data management system performs across a wide spectrum of SPARQL queries with varying structural characteristics and selectivity classes. We subsequently added streaming RDF testing capability to WatDiv working with MMath student Libo Gao and Prof. Lukasz Golab. WatDiv has its own web site here.
Relevant Projects
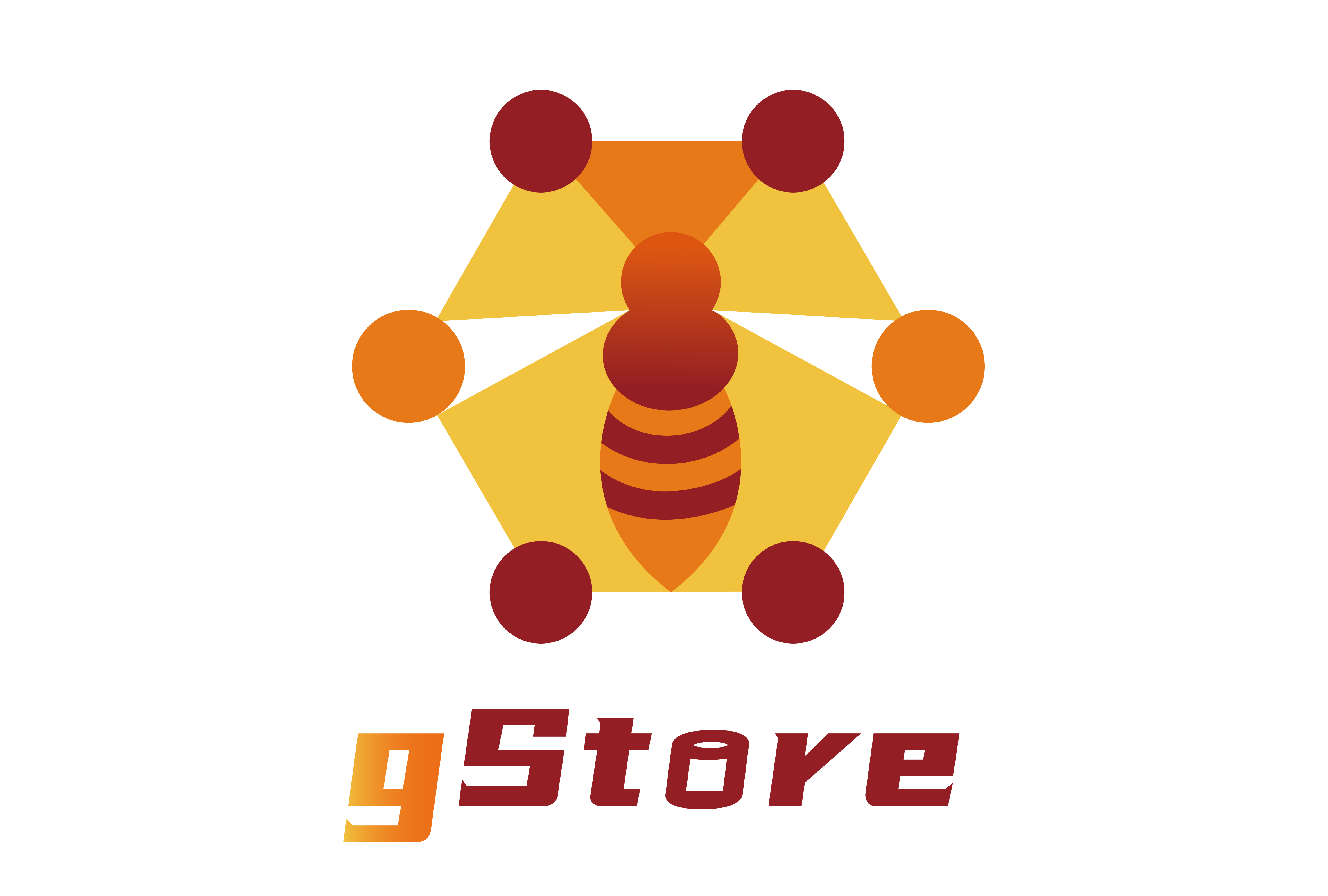
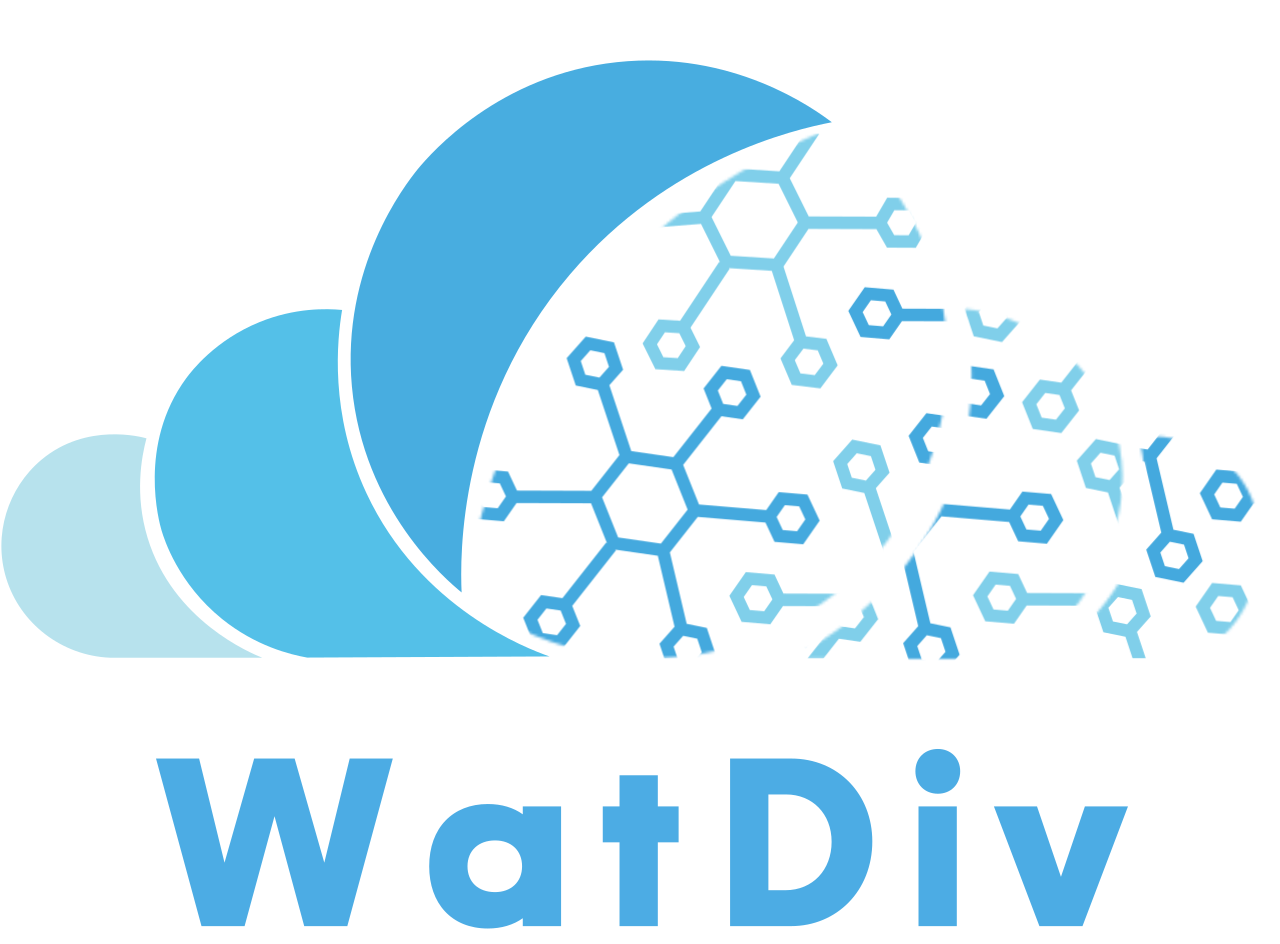
Publications
- P. Peng, M. T. Özsu, L. Zou, C. Yan, C.Liu. MPC: Minimum Property-Cut RDF Graph Partitioning. In Proc. 28th IEEE Int. Conf. on Data Eng., pages 192-204, 2022.
- P. Peng, Q. Ge, L. Zou, M. T. Özsu, Z. Xu, and D. Zhao. Optimizing Multi-Query Evaluation in Federated RDF Systems, IEEE Trans. Knowledge and Data Eng., 33(4):1692–1707, 2021.
- G. Aluç, M. T. Özsu, and K. Daudjee. Clustering RDF Databases Using Tunable-LSH, VLDB Journal, 28(2): 173-195, 2019.
- L. Gao, L. Golab, M. T. Özsu, G. Aluç. Stream WatDiv: A Streaming RDF Benchmark, In Proc. International Workshop on Semantic Big Data, pages 1-6, 2018.
- O. Hartig and M. T. Özsu. Walking without a Map: Ranking-Based Traversal for Querying Linked Data, In Proc. 15th International Semantic Web Conference, pages 305–324, 2016.
- P. Peng, L. Zou, M. T. Özsu, L. Chen, D. Zhao.Processing SPARQL Queries Over Distributed RDF Graphs, VLDB Journal, 25(2):243–268, 2016.
- G. Aluç, M. T. Özsu, K. Daudjee, and O. Hartig.Executing queries over schemaless RDF databases, In Proc. 31st Int. Conf. on Data Engineering, pages 807 - 818, 2015.
- L. Zou, M. T. Özsu, L. Chen, X. Sheng, R. Huang, and D. Zhao.gStore: A Graph-based SPARQL Query Engine, VLDB Journal, 23(4): 565-590, 2014.
- G. Aluç, O. Hartig, M. T. Özsu, and K. Daudjee. Diversified stress testing of RDF data management systems, In Proc. 13th Int. Semantic Web Conference, Part I, pages 197–212, 2014.
- G. Aluç, M. T. Özsu, and K. Daudjee. Workload matters: Why RDF databases need a new design, Proc. VLDB Endowment, 7(10):837–840, 2014.
- G. Aluç, M. T. Özsu, K. Daudjee, and O. Hartig. chameleon-db: a workload-aware robust RDF data management system,Technical Report CS-2013-10, University of Waterloo, 2013.
Other publications on this topic can be found here.
Scale-out data management
Work in this area typically overlaps with the previous two topics as I investigate how graph processing and RDF data management scale-out over distributed and parallel systems.
- With graduate students Khaled Ammar and Minyang Han, and Prof. Khuzaima Daudjee, we investigated the scale-out characteristics of graph processing systems.
- With former graduate student Dr. Anıl Paçaçı, we experimentally evaluated the behaviour of graph partitioning techniques.
- With Prof. Da Yan (University of Alabama) and his students, we have been studying scale-out solutions for executing online (light-weight) queries over very large graphs (Quegel system) and on graph mining (G-thinker system).
- More recently, I have been interested in the design of totally disaggregated distributed system design. In this work, I collaborate with Profs. Jianguo Wang and Walid Aref (Purdue University) and their students.
Publications
- R. Wang, J. Wang, S. Idreos, M.T. Özsu, W. G. Aref. The Case for Distributed Shared-Memory Databases with RDMA-Enabled Memory Disaggregation, Proc. VLDB Endowment, 16(1): 15-22, 2022.
- D. Yan, G. Guo, J. Khalil, M. T. Özsu, Wei-Shinn Ku, and John C.S. Lui. G-thinker: A general distributed framework for finding qualified subgraphs in a big graph with load balancing. VLDB Journal, 31: 287–320, 2022
- P. Valduriez, R. Jimenez-Peris, and M. T. Özsu. Distributed database systems: The case for NewSQL. In Abdelkader Hameurlain and A. Min Tjoa, editors, Transactions on Large-Scale Data- and Knowledge-Centered Systems, pages 1–15. Springer, Berlin, Heidelberg, 2021.
- A. Pacaci and M.T. Özsu. Experimental Analysis of Streaming Algorithms for Graph Partitioning, In Proc. ACM SIGMOD Int. Conf. Management of Data, pages 1375-1392, 2019.
- K. Ammar and M. T. Özsu. Experimental Analysis of Distributed Graph Systems, Proc. VLDB Endowment, 11(10): 1151-1164, 2018.
- S. Salihoglu and M. T. Özsu. Response to `Scale Up or Scale Out for Graph Processing?', IEEE Internet Comput., 22(5):18–24, 2018.
- D. Yan, J. Cheng, M. T. Özsu, F. Yang, Y. Lu, J. C.S. Liu, Q. Zhang, and W. Ng. A General-Purpose Query-Centric Framework for Querying Big Graphs. Proc. VLDB Endowment, 9(7):564 – 575, 2016.
Other publications on this topic can be found here.